Perhaps I shouldn't try to make resolutions: I resolved to blog book notes till the end of the year, and instead I'm writing something about estimation.
A power law is a relationship of the form $y = \gamma_0 x^{\gamma_1}$ and can be linearized for estimation using OLS (with a very stretchy assumption on stochastic disturbances, but let's not quibble) into
$\log(y) = \beta_0 + \beta_1 \log(x) +\epsilon$,
from which the original parameters can be trivially recovered:
$\hat\gamma_0 = \exp(\hat\beta_0)$ and $\hat\gamma_1 = \hat\beta_1$.
Power laws are plentiful in Nature, especially when one includes the degree distribution of social networks in a – generous and uncommon, I admit it – definition of Nature. An usually proposed source of power law degree distribution is preferential attachment in network formation: the probability of a new node $i$ being connected to an old node $j$ is an increasing function of the degree of $j$.
The problem with power laws in the wild is that they are really hard to estimate precisely, and I got very annoyed at the glibness of some articles, which report estimation of power laws in highly dequantized manner: they don't actually show the estimates or their descriptive statistics, only charts with no error bars.
Here's my problem: it's well-known that even small stochastic disturbances can make parameter identification in power law data very difficult. And yet, that is never mentioned in those papers. This omission, coupled with the lack of actual estimates and their descriptive statistics, is unforgivable. And suspicious.
Perhaps this needs a couple of numerical examples to clarify; as they say at the end of each season of television shows now:
– To be continued –
Non-work posts by Jose Camoes Silva; repurposed in May 2019 as a blog mostly about innumeracy and related matters, though not exclusively.
Wednesday, December 21, 2011
Tuesday, December 20, 2011
Marginalia: Writing in one's books
I've done it for a long time now, shocking behavior though it is to some of my family and friends.
WHY I make notes
Some of my family members and friends are shocked that I write in my books. The reasons to keep the books in pristine condition vary from maintaining resale value (not an issue for me, as I don't think of books as transient presences in my life) to keeping the integrity of the author's work. Obviously, if I had a first edition of Newton's Principia, I wouldn't write on in; the books I write on are workaday copies, many of them cheap paperbacks or technical books.
The reason why I makes notes is threefold:
To better understand the book as I read it. Actively reading a book, especially a non-fiction or work book, is essentially a dialog between the book and the knowledge I can access, both in my mind and in outside references. Deciding what is important enough to highlight and what points deserve further elaboration in the form of commentary or an example that I furnish, makes reading a much more immersive experience than simply processing the words.
To collect my ideas from several readings (I read many books more than once) into a place where they are not lost. Sometimes points from a previous reading are more clarifying to me than the text itself, sometimes I disagree vehemently with what I wrote before.
To refer to later when I need to find something in the book. This is particularly important in books that I read for work, in particular for technical books where many of the details have been left out (for space reasons) but I added notes that fill those in for the parts I care about.
WHAT types of notes I make
In an earlier post about marginalia on my personal blog I included this image (click for bigger),
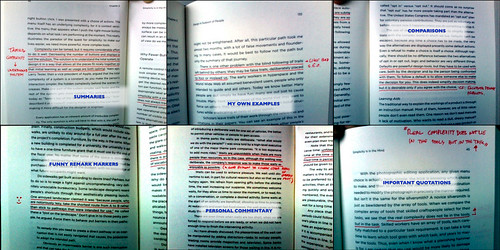
showing some notes I made while reading the book Living With Complexity, by Donald Norman. These notes fell into six cases:
Summaries of the arguments in text. Often texts will take long circuitous routes to get to the point. (Norman's book is not one of these.) I tend to write quick summaries, usually in implication form like the one above, that cut down the entropy.
My examples to complement the text. Sometimes I happen to know better examples, or examples that I prefer, than those in the book; in that case I tend to note them in the book so that the example is always connected to the context in which I thought of it. This is particularly useful in work books (and papers, of course) when I turn them into teaching or executive education materials.
Comparisons with external materials. In this case I make a note to compare Norman's point about default choices with the problems Facebook faced in similar matters regarding its privacy.
Notable passages. Marking funny passages with smiley faces and surprising passages with an exclamation point helps find these when browsing the book quickly. Occasionally I also mark passages for style or felicitous turn of phrase, typically with "nice!" on the margin.
Personal commentary. Sometimes the text provokes some reaction that I think is work recording in the book. I don't write review-like commentary in books as a general rule, but I might note something about missing or hidden assumptions, innumeracy, biases, statistical issues; I might also comment positively on an idea, for example, that I had never thought of except for the text.
Quotable passages. These are self-explanatory and particularly easy to make on eBooks. Here's one from George Orwell's Homage To Catalonia:
A few other types of marginalia that I have used in other books:
Proofs and analysis to complement what's in the text. As an example, in a PNAS paper on predictions based on search, the authors call $\log(y) = \beta_0 + \beta_1 \log(x)$ a linear model, with the logarithms used to account for the skewness of the variables. I inserted a note that this is clearly a power law relationship, not a linear relationship, with the two steps of algebra that show $y = e^{\beta_0} \times x^{\beta_1}$, in case I happen to be distracted when I reread this paper and can't think through the baby math.
Adding missing references or checking the references (which sometime are incorrect, in which case I correct them). Yep, I'm an academic nerd at heart; but these are important, like a chain of custody for evidence or the provenance records for a work of art.
Diagrams clarifying complicated points. I do this in part because I like visual thinking and in part because if I ever need to present the material to an audience I'll have a starting point for visual support design.
Data that complements the text. Sometimes the text is dequantized and refers to a story for which data is available. I find that adding the data to the story helps me get a better perspective and also if I ever want to use the story I'll have the data there to make a better case.
Counter-arguments. Sometimes I disagree with the text, or at least with the lack of feasible counter-arguments (even when I agree with a position I don't like that the author presents the opposing points of view only in strawman form), so I write the counter-arguments in order to remind me that they exist and the presentation in the text doesn't do them justice.
Markers for things that I want to get. For example, while reading Ted Gioia's The History of Jazz, I marked several recordings that he mentions for acquisition; when reading technical papers I tend to mark the references I want to check; when reading reviews I tend to add things to wishlists (though I also prune these wishlists often).
HOW to make notes
A few practical points for writing marginalia:
Highlighters are not good for long-term notes. They either darken significantly, making it hard to read the highlighted text, or they fade, losing the highlight. I prefer underlining with a high contrast color for short sentences or segments or marking beginning and end of passages on the margin.
Margins are not the only place. I add free-standing inserts, usually in the form of large Post-Its or pieces of paper. Important management tip: write the page number the note refers to on the note.
Transcribing important notes to a searchable format (a text file on my laptop) makes it easy to find stuff later. This is one of the advantages of eBooks of the various types (Kindle, iBook, O'Reilly PDFs), making it easy to search notes and highlights.
Keeping a commonplace book of felicitous turns of phrase (the ones in the books and the ones I come up with) either in a file or on an old-style paper journal helps me become a better writer.
-- -- -- --
Note: This blog may become a little more varied in topics as I decided to write posts more often to practice writing for a general audience. After all, the best way to become a better writer is to write and let others see it. (No comments on the blog, but plenty of ones by email from people I know.)
WHY I make notes
Some of my family members and friends are shocked that I write in my books. The reasons to keep the books in pristine condition vary from maintaining resale value (not an issue for me, as I don't think of books as transient presences in my life) to keeping the integrity of the author's work. Obviously, if I had a first edition of Newton's Principia, I wouldn't write on in; the books I write on are workaday copies, many of them cheap paperbacks or technical books.
The reason why I makes notes is threefold:
To better understand the book as I read it. Actively reading a book, especially a non-fiction or work book, is essentially a dialog between the book and the knowledge I can access, both in my mind and in outside references. Deciding what is important enough to highlight and what points deserve further elaboration in the form of commentary or an example that I furnish, makes reading a much more immersive experience than simply processing the words.
To collect my ideas from several readings (I read many books more than once) into a place where they are not lost. Sometimes points from a previous reading are more clarifying to me than the text itself, sometimes I disagree vehemently with what I wrote before.
To refer to later when I need to find something in the book. This is particularly important in books that I read for work, in particular for technical books where many of the details have been left out (for space reasons) but I added notes that fill those in for the parts I care about.
WHAT types of notes I make
In an earlier post about marginalia on my personal blog I included this image (click for bigger),
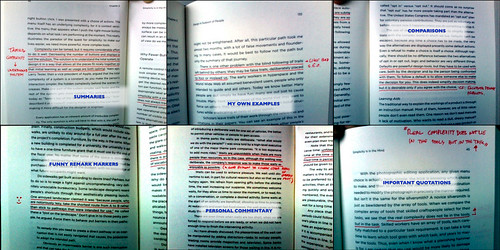
showing some notes I made while reading the book Living With Complexity, by Donald Norman. These notes fell into six cases:
Summaries of the arguments in text. Often texts will take long circuitous routes to get to the point. (Norman's book is not one of these.) I tend to write quick summaries, usually in implication form like the one above, that cut down the entropy.
My examples to complement the text. Sometimes I happen to know better examples, or examples that I prefer, than those in the book; in that case I tend to note them in the book so that the example is always connected to the context in which I thought of it. This is particularly useful in work books (and papers, of course) when I turn them into teaching or executive education materials.
Comparisons with external materials. In this case I make a note to compare Norman's point about default choices with the problems Facebook faced in similar matters regarding its privacy.
Notable passages. Marking funny passages with smiley faces and surprising passages with an exclamation point helps find these when browsing the book quickly. Occasionally I also mark passages for style or felicitous turn of phrase, typically with "nice!" on the margin.
Personal commentary. Sometimes the text provokes some reaction that I think is work recording in the book. I don't write review-like commentary in books as a general rule, but I might note something about missing or hidden assumptions, innumeracy, biases, statistical issues; I might also comment positively on an idea, for example, that I had never thought of except for the text.
Quotable passages. These are self-explanatory and particularly easy to make on eBooks. Here's one from George Orwell's Homage To Catalonia:
The constant come-and-go of troops had reduced the village to a state of unspeakable filth. It did not possess and never had possessed such a thing as a lavatory or a drain of any kind, and there was not a square yard anywhere where you could tread without watching your step. (Chapter 2.)
A few other types of marginalia that I have used in other books:
Proofs and analysis to complement what's in the text. As an example, in a PNAS paper on predictions based on search, the authors call $\log(y) = \beta_0 + \beta_1 \log(x)$ a linear model, with the logarithms used to account for the skewness of the variables. I inserted a note that this is clearly a power law relationship, not a linear relationship, with the two steps of algebra that show $y = e^{\beta_0} \times x^{\beta_1}$, in case I happen to be distracted when I reread this paper and can't think through the baby math.
Adding missing references or checking the references (which sometime are incorrect, in which case I correct them). Yep, I'm an academic nerd at heart; but these are important, like a chain of custody for evidence or the provenance records for a work of art.
Diagrams clarifying complicated points. I do this in part because I like visual thinking and in part because if I ever need to present the material to an audience I'll have a starting point for visual support design.
Data that complements the text. Sometimes the text is dequantized and refers to a story for which data is available. I find that adding the data to the story helps me get a better perspective and also if I ever want to use the story I'll have the data there to make a better case.
Counter-arguments. Sometimes I disagree with the text, or at least with the lack of feasible counter-arguments (even when I agree with a position I don't like that the author presents the opposing points of view only in strawman form), so I write the counter-arguments in order to remind me that they exist and the presentation in the text doesn't do them justice.
Markers for things that I want to get. For example, while reading Ted Gioia's The History of Jazz, I marked several recordings that he mentions for acquisition; when reading technical papers I tend to mark the references I want to check; when reading reviews I tend to add things to wishlists (though I also prune these wishlists often).
HOW to make notes
A few practical points for writing marginalia:
Highlighters are not good for long-term notes. They either darken significantly, making it hard to read the highlighted text, or they fade, losing the highlight. I prefer underlining with a high contrast color for short sentences or segments or marking beginning and end of passages on the margin.
Margins are not the only place. I add free-standing inserts, usually in the form of large Post-Its or pieces of paper. Important management tip: write the page number the note refers to on the note.
Transcribing important notes to a searchable format (a text file on my laptop) makes it easy to find stuff later. This is one of the advantages of eBooks of the various types (Kindle, iBook, O'Reilly PDFs), making it easy to search notes and highlights.
Keeping a commonplace book of felicitous turns of phrase (the ones in the books and the ones I come up with) either in a file or on an old-style paper journal helps me become a better writer.
-- -- -- --
Note: This blog may become a little more varied in topics as I decided to write posts more often to practice writing for a general audience. After all, the best way to become a better writer is to write and let others see it. (No comments on the blog, but plenty of ones by email from people I know.)
Monday, December 12, 2011
How many possible topologies can a N-node network have?
Short answer, for an undirected network: $2^{N(N-1)/2}$.
Essentially the number of edges is $N(N-1)/2$ so the number of possible topologies is two raised to the number of edges, capturing every possible case where an edge can either be present or absent. For a directed network the number of edges is twice that of those in an undirected network so the number of possible topologies is the square (or just remove the $/2$ part from the formula above).
To show how quickly things get out of control, here are some numbers:
$N=1 \Rightarrow 1$ topology
$N=2 \Rightarrow 2$ topologies
$N=3 \Rightarrow 8$ topologies
$N=4 \Rightarrow 64$ topologies
$N=5 \Rightarrow 1024$ topologies
$N=6 \Rightarrow 32,768$ topologies
$N=7 \Rightarrow 2,097,152$ topologies
$N=8 \Rightarrow 268,435,456$ topologies
$N=9 \Rightarrow 68,719,476,736$ topologies
$N=10 \Rightarrow 35,184,372,088,832$ topologies
$N=20 \Rightarrow 1.5693 \times 10^{57}$ topologies
$N=30 \Rightarrow 8.8725 \times 10^{130}$ topologies
$N=40 \Rightarrow 6.3591 \times 10^{234}$ topologies
$N=50 \Rightarrow 5.7776 \times 10^{368}$ topologies
This is the reason why any serious analysis of a network requires the use of mathematical modeling and computer processing: our human brains are not equipped to deal with this kind of exploding complexity.
And for the visual learners, here's a graph denoting the pointlessness of trying to grasp network topologies "by hand" (note logarithmic vertical scale):
Essentially the number of edges is $N(N-1)/2$ so the number of possible topologies is two raised to the number of edges, capturing every possible case where an edge can either be present or absent. For a directed network the number of edges is twice that of those in an undirected network so the number of possible topologies is the square (or just remove the $/2$ part from the formula above).
To show how quickly things get out of control, here are some numbers:
$N=1 \Rightarrow 1$ topology
$N=2 \Rightarrow 2$ topologies
$N=3 \Rightarrow 8$ topologies
$N=4 \Rightarrow 64$ topologies
$N=5 \Rightarrow 1024$ topologies
$N=6 \Rightarrow 32,768$ topologies
$N=7 \Rightarrow 2,097,152$ topologies
$N=8 \Rightarrow 268,435,456$ topologies
$N=9 \Rightarrow 68,719,476,736$ topologies
$N=10 \Rightarrow 35,184,372,088,832$ topologies
$N=20 \Rightarrow 1.5693 \times 10^{57}$ topologies
$N=30 \Rightarrow 8.8725 \times 10^{130}$ topologies
$N=40 \Rightarrow 6.3591 \times 10^{234}$ topologies
$N=50 \Rightarrow 5.7776 \times 10^{368}$ topologies
This is the reason why any serious analysis of a network requires the use of mathematical modeling and computer processing: our human brains are not equipped to deal with this kind of exploding complexity.
And for the visual learners, here's a graph denoting the pointlessness of trying to grasp network topologies "by hand" (note logarithmic vertical scale):
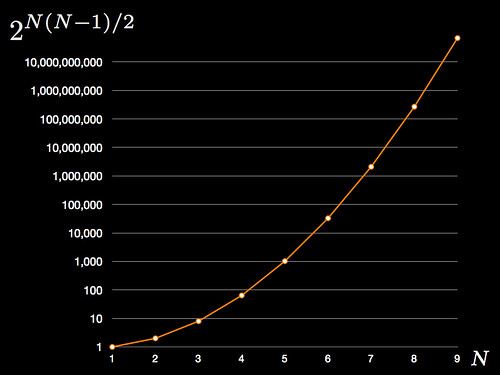
Labels:
combinatorics,
mathematics,
networks
Saturday, December 3, 2011
Why I'm not a fan of "presentation training"
Because there are too many different types of presentation for any sort of abstract training to be effective. So "presentation training" ends up – at best – being "presentation software training."
Learning about information design, writing and general verbal communication, stage management and stage presence, and operation of software and tools used in presentations may help one become a better presenter. But, like in so many technical fields, all of these need some study of the foundations followed by a lot of field- and person-specific practice.
I recommend Edward Tufte's books (and seminar) for information design; Strunk and White's The Elements of Style, James Humes's Speak like Churchill, Stand like Lincoln, and William Zinsser's On Writing Well for verbal communication; and a quick read of the manual followed by exploration of the presentation software one uses. I have no recommendations regarding stage management and stage presence short of joining a theatre group, which is perhaps too much of a commitment for most presenters.
I have already written pretty much all I think about presentation preparation; the present post is about my dislike of "presentation training." To be clear, this is not about preparation for teaching or training to be an instructor. These, being specialized skills – and typically field-specific skills – are a different case.
Problem 1: Generic presentation training is unlikely to help any but the most incompetent of presenters
Since an effective presentation is one designed for its objective, within the norms of its field, targeted to its specific audience, and using the technical knowledge of its field, what use is it to learn generic rules, beyond the minimum of information design, clarity in verbal expression, and stage presence?
(My understanding from people who have attended presentation training is that there was little about information design, nothing about verbal expression, and just platitudes about stage presence.)
For someone who knows nothing about presentations and learns the basics of operating the software, presentation training may be of some use. I think Tufte made this argument: the great presenters won't be goaded into becoming "death by powerpoint" presenters just because they use the software; the terrible presenters will be forced to come up with some talking points, which may help their presentations be less disastrous. But the rest will become worse presenters by focussing on the software and some hackneyed rules – instead of the content of and the audience for the presentation.
Problem 2: Presentation trainers tend to be clueless about the needs of technical presentations
Or, the Norman Critique of the Tufte Table Argument, writ large.
The argument (which I wrote as point 1 in this post) is essentially that looking at a table, a formula, or a diagram as a presentation object – understanding its aesthetics, its information design, its use of color and type – is very different from looking at a table to make sense of the numbers therein, understand the implications of a formula to a mathematical or chemical model, and interpret the implications of the diagram for its field.
Tufte, in his attack on Powerpoint, talks about a table but focusses on its design, not how the numbers would be used, which is what prompted Donald Norman to write his critique; but, of all the people who could be said to be involved in presentation training, Tufte is actually the strongest advocate for content.
The fact remains that there's a very big difference between technical material which is used as a prop to illustrate some presentation device or technique to an audience which is mostly outside the technical field of the material and the same material being used to make a technical point to an audience of the appropriate technical field.
Presentation training, being generic, cannot give specific rules for a given field; but those rules are actually useful to anyone in the field who has questions about how to present something.
Problem 3: Presentation training actions are typically presentations (lectures), which is not an effective way to teach technical material
The best way to teach technical material is to have the students prepare by reading the foundations (or watching video on their own, allowing them to pace the delivery by their own learning speed) and preparing for a discussion or exercise applying what they learned.
This is called participant-centered learning; it's the way people learn technical material. Even in lecture courses the actual learning only happens when the students practice the material.
Almost all presentation training is done in lecture form, delivered as a presentation from the instructor with question-and-answer periods for the audience. But since the audience doesn't actually practice the material in the lecture, they may have only questions of clarification. The real questions that appear during actual practice don't come up during a lecture, and those are the questions that really need an answer.
Problem 4: Most presentation training is too narrowly bracketed
Because it's generic, presentation training misses the point of making a presentation to begin with.
After all, presentations aren't made in a vacuum: there's a purpose to the presentation (say, report market research to decision-makers), an audience with specific needs (product designers who need to understand the parameters of the consumer choice so they can tweak the product line), supporting material that may be used for further reference (a written report with the details of the research), action items and metrics for those items (follow-up research and a schedule of deliverables and budget), and other elements that depend on the presentation.
There's also the culture of the organization which hosts the presentation, disclosure and privacy issues, reliability of sources, and a host of matters apparently unrelated to a presentation that determine its success a lot more than the design of the slides.
In fact, the use of slides, or the idea of a speaker talking to an audience, is itself a constraint on the type of presentations the training is focussed on. And that trains people to think of a presentation as a lecture-style presentation. Many presentations are interactive, perhaps with the "presenter" taking the position of moderator or arbitrator; some presentations are made in roundtable fashion, as a discussion where the main presenter is one of many voices.
Some time ago, I summarized a broader view of a specific type of presentation event (data scientists presenting results to managers) in this diagram, illustrating why and how I thought data scientists should take more care with presentation design (click for larger):
(Note that this is specific advice for people making presentations based on data analysis to managers or decision-makers that rely on the data analysis for action, but cannot do the analysis themselves. Hence the blue rules on the right to minimize the miscommunication between the people from two different fields. This is what I mean by field-specific presentation training.)
These are four reasons why I don't like generic presentation training. Really it's just one: generic presentation training assumes that content is something secondary, and that assumption is the reason why we see so many bad presentations to begin with.
NOTE: Participant-centered learning is a general term for using the class time for discussion and exercises, not necessarily for the Harvard Case Method, which is one form of participant-centered learning.
Related posts:
Posts on presentations in my personal blog.
Posts on teaching in my personal blog.
Posts on presentations in this blog.
My 3500-word post on preparing presentations.
Learning about information design, writing and general verbal communication, stage management and stage presence, and operation of software and tools used in presentations may help one become a better presenter. But, like in so many technical fields, all of these need some study of the foundations followed by a lot of field- and person-specific practice.
I recommend Edward Tufte's books (and seminar) for information design; Strunk and White's The Elements of Style, James Humes's Speak like Churchill, Stand like Lincoln, and William Zinsser's On Writing Well for verbal communication; and a quick read of the manual followed by exploration of the presentation software one uses. I have no recommendations regarding stage management and stage presence short of joining a theatre group, which is perhaps too much of a commitment for most presenters.
I have already written pretty much all I think about presentation preparation; the present post is about my dislike of "presentation training." To be clear, this is not about preparation for teaching or training to be an instructor. These, being specialized skills – and typically field-specific skills – are a different case.
Problem 1: Generic presentation training is unlikely to help any but the most incompetent of presenters
Since an effective presentation is one designed for its objective, within the norms of its field, targeted to its specific audience, and using the technical knowledge of its field, what use is it to learn generic rules, beyond the minimum of information design, clarity in verbal expression, and stage presence?
(My understanding from people who have attended presentation training is that there was little about information design, nothing about verbal expression, and just platitudes about stage presence.)
For someone who knows nothing about presentations and learns the basics of operating the software, presentation training may be of some use. I think Tufte made this argument: the great presenters won't be goaded into becoming "death by powerpoint" presenters just because they use the software; the terrible presenters will be forced to come up with some talking points, which may help their presentations be less disastrous. But the rest will become worse presenters by focussing on the software and some hackneyed rules – instead of the content of and the audience for the presentation.
Problem 2: Presentation trainers tend to be clueless about the needs of technical presentations
Or, the Norman Critique of the Tufte Table Argument, writ large.
The argument (which I wrote as point 1 in this post) is essentially that looking at a table, a formula, or a diagram as a presentation object – understanding its aesthetics, its information design, its use of color and type – is very different from looking at a table to make sense of the numbers therein, understand the implications of a formula to a mathematical or chemical model, and interpret the implications of the diagram for its field.
Tufte, in his attack on Powerpoint, talks about a table but focusses on its design, not how the numbers would be used, which is what prompted Donald Norman to write his critique; but, of all the people who could be said to be involved in presentation training, Tufte is actually the strongest advocate for content.
The fact remains that there's a very big difference between technical material which is used as a prop to illustrate some presentation device or technique to an audience which is mostly outside the technical field of the material and the same material being used to make a technical point to an audience of the appropriate technical field.
Presentation training, being generic, cannot give specific rules for a given field; but those rules are actually useful to anyone in the field who has questions about how to present something.
Problem 3: Presentation training actions are typically presentations (lectures), which is not an effective way to teach technical material
The best way to teach technical material is to have the students prepare by reading the foundations (or watching video on their own, allowing them to pace the delivery by their own learning speed) and preparing for a discussion or exercise applying what they learned.
This is called participant-centered learning; it's the way people learn technical material. Even in lecture courses the actual learning only happens when the students practice the material.
Almost all presentation training is done in lecture form, delivered as a presentation from the instructor with question-and-answer periods for the audience. But since the audience doesn't actually practice the material in the lecture, they may have only questions of clarification. The real questions that appear during actual practice don't come up during a lecture, and those are the questions that really need an answer.
Problem 4: Most presentation training is too narrowly bracketed
Because it's generic, presentation training misses the point of making a presentation to begin with.
After all, presentations aren't made in a vacuum: there's a purpose to the presentation (say, report market research to decision-makers), an audience with specific needs (product designers who need to understand the parameters of the consumer choice so they can tweak the product line), supporting material that may be used for further reference (a written report with the details of the research), action items and metrics for those items (follow-up research and a schedule of deliverables and budget), and other elements that depend on the presentation.
There's also the culture of the organization which hosts the presentation, disclosure and privacy issues, reliability of sources, and a host of matters apparently unrelated to a presentation that determine its success a lot more than the design of the slides.
In fact, the use of slides, or the idea of a speaker talking to an audience, is itself a constraint on the type of presentations the training is focussed on. And that trains people to think of a presentation as a lecture-style presentation. Many presentations are interactive, perhaps with the "presenter" taking the position of moderator or arbitrator; some presentations are made in roundtable fashion, as a discussion where the main presenter is one of many voices.
Some time ago, I summarized a broader view of a specific type of presentation event (data scientists presenting results to managers) in this diagram, illustrating why and how I thought data scientists should take more care with presentation design (click for larger):
(Note that this is specific advice for people making presentations based on data analysis to managers or decision-makers that rely on the data analysis for action, but cannot do the analysis themselves. Hence the blue rules on the right to minimize the miscommunication between the people from two different fields. This is what I mean by field-specific presentation training.)
These are four reasons why I don't like generic presentation training. Really it's just one: generic presentation training assumes that content is something secondary, and that assumption is the reason why we see so many bad presentations to begin with.
NOTE: Participant-centered learning is a general term for using the class time for discussion and exercises, not necessarily for the Harvard Case Method, which is one form of participant-centered learning.
Related posts:
Posts on presentations in my personal blog.
Posts on teaching in my personal blog.
Posts on presentations in this blog.
My 3500-word post on preparing presentations.
Labels:
presentations,
teaching
Friday, December 2, 2011
Dilbert gets the Correlation-Causation difference wrong
This was the Dilbert comic strip for Nov. 28, 2011:
It seems to imply that even though there's a correlation between the pointy-haired boss leaving Dilbert's cubicle and receiving an anonymous email about the worst boss in the world, there's no causation.
THAT IS WRONG!
Clearly there's causation: PHB leaves Dilbert's cubicle, which causes Wally to send the anonymous email. PHB's implication that he thinks Dilbert sends the email is wrong, but that doesn't mean that the correlation he noticed isn't in this case created by a causal link between leaving Dilbert's cubicle and getting the email.
I think Edward Tufte once said that the statement "correlation is not causation" was incomplete; at least it should read "correlation is not causation, but it sure hints at some relationship that must be investigated further." Or words to that effect.
It seems to imply that even though there's a correlation between the pointy-haired boss leaving Dilbert's cubicle and receiving an anonymous email about the worst boss in the world, there's no causation.
THAT IS WRONG!
Clearly there's causation: PHB leaves Dilbert's cubicle, which causes Wally to send the anonymous email. PHB's implication that he thinks Dilbert sends the email is wrong, but that doesn't mean that the correlation he noticed isn't in this case created by a causal link between leaving Dilbert's cubicle and getting the email.
I think Edward Tufte once said that the statement "correlation is not causation" was incomplete; at least it should read "correlation is not causation, but it sure hints at some relationship that must be investigated further." Or words to that effect.
Labels:
causality,
Dilbert,
Probability,
statistics
Friday, November 25, 2011
Online Education and the Dentist vs Personal Trainer Models of Learning
I'm a little skeptical about online education. About 2/3 skeptical.
Most of the (traditional) teaching I received was squarely based on what I call the Dentist Model of Education: a [student|patient] goes into the [classroom|dentist's office] and the [instructor|dentist] does something technical to the [student|patient]. Once the professional is done, the [student|patient] goes away and [forgets the lecture|never flosses].
I learned almost nothing from that teaching. Like every other person in a technical field, I learned from studying and solving practice problems. (Rule of thumb: learning is 1% lecture, 9% study, 90% practice problems.)
A better education model, the Personal Trainer Model of Education asserts that, like in fitness training, results come from the [trainee|student] practicing the [movements|materials] himself/herself. The job of the [personal trainer|instructor] is to guide that practice and select [exercises|materials] that are appropriate to the [training|instruction] objectives.
Which is why I'm two-thirds skeptical of the goodness of online education.
Obviously there are advantages to online materials: there's low distribution cost, which allows many people to access high quality materials; there's a culture of sharing educational materials, spearheaded by some of the world's premier education institutions; there are many forums, question and answer sites and – for those willing to pay a small fee – actual online courses with instructors and tests.
Leaving aside the broad accessibility of materials, there's no getting around the 1-9-90 rule for learning. Watching Walter Lewin teaching physics may be entertaining, but without practicing, by solving problem sets, no one watching will become a physicist.
Consider the plethora of online personal training advice and assume that the aspiring trainee manages to find a trainer who knows what he/she is doing. Would this aspiring trainee get better at her fitness exercises by reading a web site and watching videos of the personal trainer exercising? And yet some people believe that they can learn computer programming by watching online lectures. (Or offline lectures, for that matter.*)
If practice is the key to success, why do so many people recognize the absurdity of the video-watching, gym-avoiding fitness trainee while at the same time assume that online lectures are the solution to technical education woes?
(Well-designed online instruction programs are much more than lectures, of course; but what most people mean by online education is not what I consider well-designed and typically is an implementation of the dentist model of education.)
The second reason why I'm skeptic (hence the two-thirds share of skepticism) is that the education system has a second component, beyond instruction: it certifies skills and knowledge. (We could debate how well it does this, but certification is one of the main functions of education institutions.)
Certification of a specific skill can be done piecemeal but complex technical fields depend on more than a student knowing the individual skills of the field; they require the ability to integrate across different sub-disciplines, to think like a member of the profession, to actually do things. That's why engineering students have engineering projects, medical students actually treat patients, etc. These are part of the certification process, which is very hard to do online or with short in-campus events, even if we remove questions of cheating from the mix.
There's enormous potential in online education, but it can only be realized by accepting that education is not like a visit to the dentist but rather like a training session at the gym. And that real, certified learning requires a lot of interaction between the education provider and the student: not something like the one-way lectures one finds online.
(This is not to say that there aren't some good online education programs, but they tend to be uncommon.)
Just like the best-equipped gym in the world will do nothing for a lazy trainee, the best online education platform in the world will do nothing for an unmotivated student. But a motivated kid with nothing but a barbell & plates can become a competitive powerlifter and a motivated kid a with a textbook will learn more than the hordes who watch online lectures while tweeting and facebooking.
The key success factor is not technology; it's the student. It always is.
ADDENDUM (Nov 27, 2011): I've received some comments to the effect that I'm just defending universities from the disruptive innovation of entrants. Perhaps, but:
Universities have several advantages over new institutions, especially when so many of these new institutions have no understanding of what technical education requires. If there was a new online way to sell hamburgers would it surprise anyone that McDs and BK were better at doing it than people who are great at online selling engineering but who never made an hamburger in their lives?
This is not to say that there isn't [vast] room to improve in both the online and offline offerings of universities. But it takes a massive dose of arrogance to assume that everything that went before (in regards to education) can be ignored because of a low cost of content distribution.
--------
* For those who never learned computer programming: you learn by writing programs and testing them. Many many many programs and many many many tests. A quick study of the basics of the language in question is necessary, but better done individually than in a lecture room. Sometimes the learning process can be jump-started by adapting other people's programs. A surefire way to not learn how to program is to listen to someone else talk about programming.
Most of the (traditional) teaching I received was squarely based on what I call the Dentist Model of Education: a [student|patient] goes into the [classroom|dentist's office] and the [instructor|dentist] does something technical to the [student|patient]. Once the professional is done, the [student|patient] goes away and [forgets the lecture|never flosses].
I learned almost nothing from that teaching. Like every other person in a technical field, I learned from studying and solving practice problems. (Rule of thumb: learning is 1% lecture, 9% study, 90% practice problems.)
A better education model, the Personal Trainer Model of Education asserts that, like in fitness training, results come from the [trainee|student] practicing the [movements|materials] himself/herself. The job of the [personal trainer|instructor] is to guide that practice and select [exercises|materials] that are appropriate to the [training|instruction] objectives.
Which is why I'm two-thirds skeptical of the goodness of online education.
Obviously there are advantages to online materials: there's low distribution cost, which allows many people to access high quality materials; there's a culture of sharing educational materials, spearheaded by some of the world's premier education institutions; there are many forums, question and answer sites and – for those willing to pay a small fee – actual online courses with instructors and tests.
Leaving aside the broad accessibility of materials, there's no getting around the 1-9-90 rule for learning. Watching Walter Lewin teaching physics may be entertaining, but without practicing, by solving problem sets, no one watching will become a physicist.
Consider the plethora of online personal training advice and assume that the aspiring trainee manages to find a trainer who knows what he/she is doing. Would this aspiring trainee get better at her fitness exercises by reading a web site and watching videos of the personal trainer exercising? And yet some people believe that they can learn computer programming by watching online lectures. (Or offline lectures, for that matter.*)
If practice is the key to success, why do so many people recognize the absurdity of the video-watching, gym-avoiding fitness trainee while at the same time assume that online lectures are the solution to technical education woes?
(Well-designed online instruction programs are much more than lectures, of course; but what most people mean by online education is not what I consider well-designed and typically is an implementation of the dentist model of education.)
The second reason why I'm skeptic (hence the two-thirds share of skepticism) is that the education system has a second component, beyond instruction: it certifies skills and knowledge. (We could debate how well it does this, but certification is one of the main functions of education institutions.)
Certification of a specific skill can be done piecemeal but complex technical fields depend on more than a student knowing the individual skills of the field; they require the ability to integrate across different sub-disciplines, to think like a member of the profession, to actually do things. That's why engineering students have engineering projects, medical students actually treat patients, etc. These are part of the certification process, which is very hard to do online or with short in-campus events, even if we remove questions of cheating from the mix.
There's enormous potential in online education, but it can only be realized by accepting that education is not like a visit to the dentist but rather like a training session at the gym. And that real, certified learning requires a lot of interaction between the education provider and the student: not something like the one-way lectures one finds online.
(This is not to say that there aren't some good online education programs, but they tend to be uncommon.)
Just like the best-equipped gym in the world will do nothing for a lazy trainee, the best online education platform in the world will do nothing for an unmotivated student. But a motivated kid with nothing but a barbell & plates can become a competitive powerlifter and a motivated kid a with a textbook will learn more than the hordes who watch online lectures while tweeting and facebooking.
The key success factor is not technology; it's the student. It always is.
ADDENDUM (Nov 27, 2011): I've received some comments to the effect that I'm just defending universities from the disruptive innovation of entrants. Perhaps, but:
Universities have several advantages over new institutions, especially when so many of these new institutions have no understanding of what technical education requires. If there was a new online way to sell hamburgers would it surprise anyone that McDs and BK were better at doing it than people who are great at online selling engineering but who never made an hamburger in their lives?
This is not to say that there isn't [vast] room to improve in both the online and offline offerings of universities. But it takes a massive dose of arrogance to assume that everything that went before (in regards to education) can be ignored because of a low cost of content distribution.
--------
* For those who never learned computer programming: you learn by writing programs and testing them. Many many many programs and many many many tests. A quick study of the basics of the language in question is necessary, but better done individually than in a lecture room. Sometimes the learning process can be jump-started by adapting other people's programs. A surefire way to not learn how to program is to listen to someone else talk about programming.
Labels:
education
Thursday, November 24, 2011
Data cleaning or cherry-picking?
Sometimes there's a fine line between data cleaning and cherry-picking your data.
My new favorite example of this is based on something Nassim Nicholas Taleb said at a talk at Penn (starting at 32 minutes in): that 92% of all kurtosis for silver in the last 40 years of trading could be traced to a single day; 83% of stock market kurtosis could also be traced to one day in 40 years.
One day in forty years is about 1/14,600 of all data. Such a disproportionate effect might lead some "outlier hunters" to discard that one data point. After all, there are many data butchers (not scientists if they do this) who create arbitrary rules for outlier detection (say, more than four standard deviations away from the mean) and use them without thinking.
In the NNT case, however, that would be counterproductive: the whole point of measuring kurtosis (or, in his argument, the problem that kurtosis is not measurable in any practical way) is to hedge against risk correctly. Underestimating kurtosis will create ineffective hedges, so disposing of the "outlier" will undermine the whole point of the estimation.
In a recent research project I removed one data point from the analysis, deeming it an outlier. But I didn't do it because it was four standard deviations from the mean alone. I found it because it did show an aggregate behavior that was five standard deviations higher than the mean. Then I examined the disaggregate data and confirmed that this was anomalous behavior: the experimental subject had clicked several times on links and immediately clicked back, not even looking at the linked page. This temporally disaggregate behavior, not the aggregate measure of total clicks, was the reason why I deemed the datum an outlier, and excluded it from analysis.
Data cleaning is an important step in data analysis. We should take care to ensure that it's done correctly.
My new favorite example of this is based on something Nassim Nicholas Taleb said at a talk at Penn (starting at 32 minutes in): that 92% of all kurtosis for silver in the last 40 years of trading could be traced to a single day; 83% of stock market kurtosis could also be traced to one day in 40 years.
One day in forty years is about 1/14,600 of all data. Such a disproportionate effect might lead some "outlier hunters" to discard that one data point. After all, there are many data butchers (not scientists if they do this) who create arbitrary rules for outlier detection (say, more than four standard deviations away from the mean) and use them without thinking.
In the NNT case, however, that would be counterproductive: the whole point of measuring kurtosis (or, in his argument, the problem that kurtosis is not measurable in any practical way) is to hedge against risk correctly. Underestimating kurtosis will create ineffective hedges, so disposing of the "outlier" will undermine the whole point of the estimation.
In a recent research project I removed one data point from the analysis, deeming it an outlier. But I didn't do it because it was four standard deviations from the mean alone. I found it because it did show an aggregate behavior that was five standard deviations higher than the mean. Then I examined the disaggregate data and confirmed that this was anomalous behavior: the experimental subject had clicked several times on links and immediately clicked back, not even looking at the linked page. This temporally disaggregate behavior, not the aggregate measure of total clicks, was the reason why I deemed the datum an outlier, and excluded it from analysis.
Data cleaning is an important step in data analysis. We should take care to ensure that it's done correctly.
Labels:
analytics,
data processing,
Probability
Sunday, November 13, 2011
Vanity Fair bungles probability example
There's an interesting article about Danny Kahneman in Vanity Fair, written by Michael Lewis. Kahneman's book Thinking: Fast And Slow is an interesting review of the state of decision psychology and well worth reading, as it the Vanity Fair article.
But the quiz attached to that article is an example of how not to popularize technical content.
This example, question 2, is wrong:
$p(math|eng) = 0.5$; half the engineers have math as a hobby.
$p(math|law) = 0.001$; one in a thousand lawyers has math as a hobby.
Then the posterior probabilities (once the description is known) are given by
I understand the representativeness heuristic, which mistakes $p(math|eng)/p(math|law)$ for $p(eng|math)/p(law|math)$, ignoring the base rates, but there's no reason to give up the inference process if some data in the description is actually informative.
-- -- -- --
* This example shows the elucidative power of working through some numbers. One might be tempted to say "ok, there's some updating, but it will probably still fall under the 10-40pct category" or "you may get large numbers with a disproportionate example like one-half of the engineers and one-in-a-thousand lawyers, but that's just an extreme case." Once we get some numbers down, these two arguments fail miserably.
Numbers are like examples, personas, and prototypes: they force assumptions and definitions out in the open.
But the quiz attached to that article is an example of how not to popularize technical content.
This example, question 2, is wrong:
A team of psychologists performed personality tests on 100 professionals, of which 30 were engineers and 70 were lawyers. Brief descriptions were written for each subject. The following is a sample of one of the resulting descriptions:
Jack is a 45-year-old man. He is married and has four children. He is generally conservative, careful, and ambitious. He shows no interest in political and social issues and spends most of his free time on his many hobbies, which include home carpentry, sailing, and mathematics.
What is the probability that Jack is one of the 30 engineers?No. Most people have knowledge beyond what is in the description; so, starting from the appropriate prior probabilities, $p(law) = 0.7$ and $p(eng) = 0.3$, they update them with the fact that engineers like math more than lawyers, $p(math|eng) >> p(math|law)$. For illustration consider
A. 10–40 percent
B. 40–60 percent
C. 60–80 percent
D. 80–100 percent
If you answered anything but A (the correct response being precisely 30 percent), you have fallen victim to the representativeness heuristic again, despite having just read about it.
$p(math|eng) = 0.5$; half the engineers have math as a hobby.
$p(math|law) = 0.001$; one in a thousand lawyers has math as a hobby.
Then the posterior probabilities (once the description is known) are given by
$p(eng|math) = \frac{ p(math|eng) \times p(eng)}{p(math)}$
$p(law|math) = \frac{ p(math|law) \times p(law)}{p(math)}$with $p(math) = p(math|eng) \times p(eng) + p(math|law) \times p(law)$. In other words, with the conditional probabilities above,
$p(eng|math) = 0.995$
$p(law|math) = 0.005$
Note that even if engineers as a rule don't like math, only a small minority does, the probability is still much higher than 0.30 as long as the minority of engineers is larger than the minority of lawyers*:
Yes, that last case is a two-to-one ratio of engineers who like math to lawyers who like math; and it still falls out of the 10-40pct category.$p(math|eng) = 0.25$ implies $p(eng|math) = 0.991$
$p(math|eng) = 0.10$ implies $p(eng|math) = 0.977$
$p(math|eng) = 0.05$ implies $p(eng|math) = 0.955$
$p(math|eng) = 0.01$ implies $p(eng|math) = 0.811$
$p(math|eng) = 0.005$ implies $p(eng|math) = 0.682$
$p(math|eng) = 0.002$ implies $p(eng|math) = 0.462$
I understand the representativeness heuristic, which mistakes $p(math|eng)/p(math|law)$ for $p(eng|math)/p(law|math)$, ignoring the base rates, but there's no reason to give up the inference process if some data in the description is actually informative.
-- -- -- --
* This example shows the elucidative power of working through some numbers. One might be tempted to say "ok, there's some updating, but it will probably still fall under the 10-40pct category" or "you may get large numbers with a disproportionate example like one-half of the engineers and one-in-a-thousand lawyers, but that's just an extreme case." Once we get some numbers down, these two arguments fail miserably.
Numbers are like examples, personas, and prototypes: they force assumptions and definitions out in the open.
Tuesday, November 1, 2011
Less
I found a magic word and it's "less."
On September 27, 2011, I decided to run a lifestyle experiment. Nothing radical, just a month of no non-essential purchases, the month of October 2011. These are the lessons from that experiment.
Separate need, want, and like
One of the clearest distinctions a "no non-essential purchases" experiment required me to make was the split between essential and non-essential.
Things like food, rent, utilities, gym membership, Audible, and Netflix I categorized as essential, or needs. The first three for obvious reasons, the last three because the hassle of suspending them wasn't worth the savings.
A second category of purchases under consideration was wants, things that I felt that I needed but could postpone the purchase until the end of the month. This included things like Steve Jobs's biography, for example. I just collected these in the Amazon wish list.
A third category was likes. Likes were things that I wanted to have but knew that I could easily live without them. (Jobs's biography doesn't fall into this category, as anyone who wants to discuss the new economy seriously has to read it. It's a requirement of my work, as far as I am concerned.) I placed these in the Amazon wish list as well.
Over time, some things that I perceived as needs were revealed as simply wants or even likes. And many wants ended up as likes. This means that just by delaying the decision to purchase for some time I made better decisions.
This doesn't mean that I won't buy something because I like it (I do have a large collection of music, art, photography, history, science, and science fiction books, all of which are not strictly necessary). What it means is that the decision to buy something is moderated by the preliminary categorization into these three levels of priority.
A corollary of this distinction is that it allows me to focus on what is really important in the activities that I engage in. I summarized some results in the following table (click for bigger):
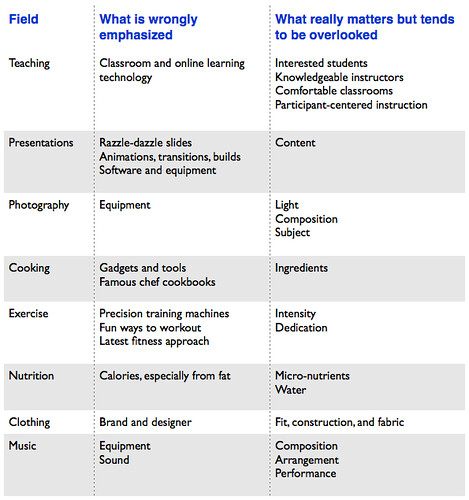
One of the regularities of this table is that the entries in the middle column (things that are wrongly emphasized) tend to be things that are bought, while entries in the last column (what really matters) tend to be things that are learned or experienced.
Correct accounting focusses on time, not on nominal money
Ok, so I can figure out a way to spend less in things that are not that necessary. Why is this a source of happiness?
Because money to spend costs time and I don't even get all the money.
When I spend one hour working a challenging technical marketing problem for my own enjoyment, I get the full benefit of that one hour of work, in the happiness solving a puzzle always brings me. When I work for one hour on something that I'd rather not be doing for a payment of X dollars, I get to keep about half of those X dollars (when everything is accounted for). I wrote an illustration of this some time ago.
In essence, money to spend comes, at least partially from doing things you'd rather not do, or doing them at times when you'd rather be doing something else, or doing them at locations that you'd rather not travel to. I like the teaching and research parts of my job, but there are many other parts that I do because it's the job. I'm lucky in that I like my job; but even so I don't like all the activities it involves.
The less money I need, the fewer additional things I have to do for money. And, interestingly, the higher my price for doing those things. (If my marginal utility of money is lower, you need to pay more for me to incur the disutility of teaching that 6-9AM on-location exec-ed seminar than you'd have to pay to a alternate version of me that really wants money to buy the latest glued "designer" suit.)
Clarity of purpose, not simply frugality, is the key aspect
I'm actually quite frugal, having never acquired the costly luxury items of a wife and children, but the lessons here are not about frugality, rather about clarity of purpose.
I have a $\$$2000 17mm ultra-wide angle tilt-shift lens on my wishlist, as a want. I do want to buy it, though I don't need it for now. Once I'm convinced that the lens on the camera, rather than my skills as a photographer, is the binding constraint in my photography, I plan to buy the lens. (Given the low speed at which my photography skill is improving, this may be a non-issue. ☺)
Many of our decisions are driven by underlying identity or symbolic reasons; other decisions are driven by narrowly framed problems; some decisions are just herd behavior or influenced by information cascades that overwhelm reasonable criteria; others still are purely hedonic, in-the-moment, impulses. Clarity of purpose avoids all these. I ask:
Why am I doing this, really?
I was surprised at how many times the answer was "erm...I don't know," "isn't everybody?" or infinitely worse "to impress X." These were not reasonable criteria for a decision. (Note that this is not just about purchase decisions, it's about all sorts of little decisions one makes every day, which deplete our wallets but also our energy, time, and patience.)
Clarity of purpose is hard to achieve during normal working hours, shopping, or the multiple activities that constitute a lifestyle. Borrowing some tools designed for lifestyle marketing, I have a simple way to do a "personal lifestyle review" using the real person "me" as the persona used in lifestyle marketing analysis. Adapted from the theory, it is:
1. Create a comprehensive list of stuff (not just material possessions, but relationships, work that is pending, even persons in one's life).
2. Associate the each entry in the stuff to a sub-persona (for non-marketers this means to a part of the lifestyle that is more or less independent of the others).
3. For each sub-persona, determine the activities which have given origin to the stuff.
4. Evaluate the activities using the "clarity of purpose" criterion: why am I doing this?
5. Purge the activities that are purely symbolic and those that were adopted for hedonic reasons but do not provide the hedonic rewards associated with their cost (in money, constraints to life, time, etc), plus any functional activities that are no longer operative.
6. Guide life decisions by the activities that survive the purge. Revise criteria only by undergoing a lifestyle review process, not by spur-of-the-moment impulses.
(This procedure is offered with no guarantees whatsoever; marketers may recognize the underlying structure from lifestyle marketing frameworks with all the consumer decisions reversed.)
Less. It works for me.
A final, cautionary thought: if the ideas I wrote here were widely adopted, most economies would crash. But I don't think there's any serious risk of that.
On September 27, 2011, I decided to run a lifestyle experiment. Nothing radical, just a month of no non-essential purchases, the month of October 2011. These are the lessons from that experiment.
Separate need, want, and like
One of the clearest distinctions a "no non-essential purchases" experiment required me to make was the split between essential and non-essential.
Things like food, rent, utilities, gym membership, Audible, and Netflix I categorized as essential, or needs. The first three for obvious reasons, the last three because the hassle of suspending them wasn't worth the savings.
A second category of purchases under consideration was wants, things that I felt that I needed but could postpone the purchase until the end of the month. This included things like Steve Jobs's biography, for example. I just collected these in the Amazon wish list.
A third category was likes. Likes were things that I wanted to have but knew that I could easily live without them. (Jobs's biography doesn't fall into this category, as anyone who wants to discuss the new economy seriously has to read it. It's a requirement of my work, as far as I am concerned.) I placed these in the Amazon wish list as well.
Over time, some things that I perceived as needs were revealed as simply wants or even likes. And many wants ended up as likes. This means that just by delaying the decision to purchase for some time I made better decisions.
This doesn't mean that I won't buy something because I like it (I do have a large collection of music, art, photography, history, science, and science fiction books, all of which are not strictly necessary). What it means is that the decision to buy something is moderated by the preliminary categorization into these three levels of priority.
A corollary of this distinction is that it allows me to focus on what is really important in the activities that I engage in. I summarized some results in the following table (click for bigger):
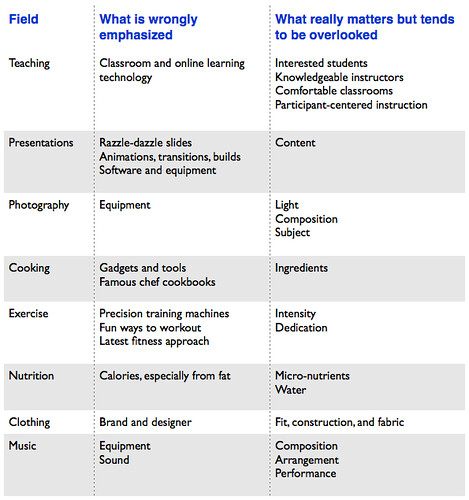
One of the regularities of this table is that the entries in the middle column (things that are wrongly emphasized) tend to be things that are bought, while entries in the last column (what really matters) tend to be things that are learned or experienced.
Correct accounting focusses on time, not on nominal money
Ok, so I can figure out a way to spend less in things that are not that necessary. Why is this a source of happiness?
Because money to spend costs time and I don't even get all the money.
When I spend one hour working a challenging technical marketing problem for my own enjoyment, I get the full benefit of that one hour of work, in the happiness solving a puzzle always brings me. When I work for one hour on something that I'd rather not be doing for a payment of X dollars, I get to keep about half of those X dollars (when everything is accounted for). I wrote an illustration of this some time ago.
In essence, money to spend comes, at least partially from doing things you'd rather not do, or doing them at times when you'd rather be doing something else, or doing them at locations that you'd rather not travel to. I like the teaching and research parts of my job, but there are many other parts that I do because it's the job. I'm lucky in that I like my job; but even so I don't like all the activities it involves.
The less money I need, the fewer additional things I have to do for money. And, interestingly, the higher my price for doing those things. (If my marginal utility of money is lower, you need to pay more for me to incur the disutility of teaching that 6-9AM on-location exec-ed seminar than you'd have to pay to a alternate version of me that really wants money to buy the latest glued "designer" suit.)
Clarity of purpose, not simply frugality, is the key aspect
I'm actually quite frugal, having never acquired the costly luxury items of a wife and children, but the lessons here are not about frugality, rather about clarity of purpose.
I have a $\$$2000 17mm ultra-wide angle tilt-shift lens on my wishlist, as a want. I do want to buy it, though I don't need it for now. Once I'm convinced that the lens on the camera, rather than my skills as a photographer, is the binding constraint in my photography, I plan to buy the lens. (Given the low speed at which my photography skill is improving, this may be a non-issue. ☺)
Many of our decisions are driven by underlying identity or symbolic reasons; other decisions are driven by narrowly framed problems; some decisions are just herd behavior or influenced by information cascades that overwhelm reasonable criteria; others still are purely hedonic, in-the-moment, impulses. Clarity of purpose avoids all these. I ask:
Why am I doing this, really?
I was surprised at how many times the answer was "erm...I don't know," "isn't everybody?" or infinitely worse "to impress X." These were not reasonable criteria for a decision. (Note that this is not just about purchase decisions, it's about all sorts of little decisions one makes every day, which deplete our wallets but also our energy, time, and patience.)
Clarity of purpose is hard to achieve during normal working hours, shopping, or the multiple activities that constitute a lifestyle. Borrowing some tools designed for lifestyle marketing, I have a simple way to do a "personal lifestyle review" using the real person "me" as the persona used in lifestyle marketing analysis. Adapted from the theory, it is:
1. Create a comprehensive list of stuff (not just material possessions, but relationships, work that is pending, even persons in one's life).
2. Associate the each entry in the stuff to a sub-persona (for non-marketers this means to a part of the lifestyle that is more or less independent of the others).
3. For each sub-persona, determine the activities which have given origin to the stuff.
4. Evaluate the activities using the "clarity of purpose" criterion: why am I doing this?
5. Purge the activities that are purely symbolic and those that were adopted for hedonic reasons but do not provide the hedonic rewards associated with their cost (in money, constraints to life, time, etc), plus any functional activities that are no longer operative.
6. Guide life decisions by the activities that survive the purge. Revise criteria only by undergoing a lifestyle review process, not by spur-of-the-moment impulses.
(This procedure is offered with no guarantees whatsoever; marketers may recognize the underlying structure from lifestyle marketing frameworks with all the consumer decisions reversed.)
Less. It works for me.
A final, cautionary thought: if the ideas I wrote here were widely adopted, most economies would crash. But I don't think there's any serious risk of that.
Labels:
lifestyle
Monday, October 24, 2011
Thinking skill, subject matter expertise, and information
Good thinking depends on all three, but they have different natures.
To illustrate, I'm going to use a forecasting tool called Scenario Planning to determine my chances of dating Milla Jovovich.
First we must figure out the causal structure of the scenario. The desired event, "Milla and I live happily ever after," we denote by $M$. Using my subject matter expertise on human relationships, I postulate that $M$ depends on a conjunction of two events:
- Event $P$ is "Paul Anderson – her husband – runs away with a starlet from one of his movies"
- Event $H$ is "I pick up the pieces of Milla's broken heart"
So the scenario can be described by $P \wedge H \Rightarrow M$. And probabilistically,
$\Pr(M) = \Pr(P) \times \Pr(H).$
Now we can use information from the philandering of movie directors and the knight-in-shining-armor behavior of engineering/business geeks [in Fantasyland, where Milla and I move in the same circles] to estimate $\Pr(P) =0.2$ (those movie directors are scoundrels) and $\Pr(H)=0.1$ (there are other chivalrous nerds willing to help Milla) for a final result of $\Pr(M)=0.02$, or 2% chance.
Of course, scenario planning allows for more granularity and for sensitivity analysis.
We could decompose event $P$ further into a conjunction of two events, $S$ for "attractive starlet in Paul's movies" and $I$ for "Paul goes insane and chooses starlet over Milla." We could now determine $\Pr(P)$ from these events instead of estimating it directly at 0.2 from the marital unreliability of movie directors in general, using $\Pr(P) = \Pr(S) \times \Pr(I).$
Or, going in another direction, we could do a sensitivity analysis. Instead of assuming a single value for $\Pr(P)$ and $\Pr(H)$, we could find upper and lower bounds, say $0.1 < \Pr(P) < 0.3$ and $0.05 < \Pr(H) < 0.15$. This would mean that $0.005 < \Pr(M) < 0.045$.
Of course, if instead of the above interpretation we had
- Event $P$ is "contraction in the supply of carbon fiber"
- Event $H$ is "increase in the demand for lightweight camera tripods and monopods"
- Event $M$ is "precipitous increase in price and shortages of carbon fiber tennis rackets"
the same scenario planning would be used for logistics management of a sports retailer provisioning.
Which brings us to the three different competencies needed for scenario planning, and more generally, for thinking about something:
Thinking skill is, in this case, knowing how to use scenarios for planning. It includes knowing that the tool exists, knowing what its strengths and weaknesses are, how to compute the final probabilities, how to do sensitivity analysis, and other procedural matters. All the computations above, which don't depend on what the events mean are pure thinking skill.
Subject matter expertise is where the specific elements of the scenario and their chains of causality come from. It includes knowing what to include and what to ignore, understanding how the various events in a specific subject area are related, and understanding the meaning of the events (as opposed to just computing inferential chains like an algorithm). So knowing that movie directors tend to abandon their wives for starlets allows me to decompose the event $P$ into $S$ and $I$ in the Milla example. But only an expert in the carbon fiber market would know how to decompose $P$ when it becomes the event "contraction in the supply of carbon fiber."
Information, in this case, are the probabilities used as inputs for calculation, as long as those probabilities come from data. (Some of these, of course, could be parameters of the scenario, which would make them subject matter expertise. Also, instead of a strict implication we could have probabilistic causality.) For example, the $\Pr(P)=0.2$ could be a simple statistical count of how many directors married to fashion models leave their wives for movie starlets.
Of these three competencies, thinking skill is the most transferrable: knowing how to do the computations associated with scenario planning allows one to do them in military forecasting or in choice of dessert for dinner. It is also one that should be carefully learned and practiced in management programs but typically is not given the importance its real-world usefulness would imply.
Subject matter expertise is the hardest to acquire – and the most valuable – since it requires both acquiring knowledge and developing judgment. It is also very hard to transfer: understanding the reactions of retailers in a given area doesn't transfer easily to forecasting nuclear proliferation.
Information is problem-specific and though it may cost money it doesn't require either training (like thinking skill) or real learning (like subject matter expertise). Knowing which information to get requires both thinking skill and subject matter expertise, of course.
Getting these three competencies confused leads to hilarious (or tragic) choices of decision-maker. For example, the idea that "smart is what matters" in recruiting for specific tasks ignores the importance of subject matter expertise.*
Conversely, sometimes a real subject matter expert makes a fool of himself when he tries to opine on matters beyond his expertise, even ones that are simple. That's because he may be very successful in his area due to the expertise making up for faulty reasoning skills, but in areas where he's not an expert those faults in reasoning skill become apparent.
Let's not pillory a deceased equine by pointing out the folly of making decisions without information; on the other hand, it's important to note the idiocy of mistaking someone who is well-informed (and just that) for a clear thinker or a knowledgeable expert.
Understanding the structure of good decisions requires separating these three competencies. It's a pity so few people do.
-- -- -- --
* "Smart" is usually a misnomer: people identified as "smart" tend to be good thinkers, not necessarily those who score highly on intelligence tests. Think of intelligence as raw strength and thinking as olympic weightlifting: the first helps the second, but strength without skill is irrelevant. In fact, some intelligent people end up being poor thinkers because they use their intelligence to defend points of view that they adopted without thinking and turned out to be seriously flawed.
Note 1: This post was inspired by a discussion about thinking and forecasting with a real clear thinker and also a subject matter expert on thinking, Wharton professor Barbara Mellers.
Note 2: No, I don't believe I have a 2% chance of dating Milla Jovovich. I chose that example precisely because it's so far from reality that it will give a smile to any of my friends or students reading this.
Labels:
business,
Decision-Science,
management,
thinking
Saturday, October 15, 2011
The costly consequences of misunderstanding cost
Apparently there's growing scarcity of some important medicines. And why wouldn't there be?
Some of these medicines are off-patent, some are price-controlled (at least in most of the world), some are bought at "negotiated" prices where one of the parties negotiating (the government) has the power to expropriate the patent from the producer. In other words, their prices are usually set at variable cost plus a small markup.
Hey, says Reggie the regulator, they're making a profit on each pill, so they should produce it anyway.
(Did you spot the error?)
(Wait for it...)
(Got it yet?)
Dear Reggie: pills are made in these things called "laboratories," that are really factories. Factories, you may be interested to know, have something called "capacity constraints," which means that using a production line for making one type of pill precludes that production line from making a different kind of pill. Manufacturers are in luck, though, because most production lines can be repurposed from one medication to another with relatively small configuration cost.
Companies make their decisions based on opportunity costs, not just variable costs. If they have a margin of say 90 cents/pill for growing longer eyelashes (I'm not kidding, there's a "medication" for that) and say 5 cents/pill to cure TB, they are going to dedicate as much of their production capacity to the eyelash-elongating "medication" as they can.* (They won't stop making the TB medication altogether because that would be bad for public relations.)
Funny how these things work, huh?
-----------
* Unless they can make more than eighteen times more TB pills than eyelash "medicine" pills with the same production facilities, of course.
Some of these medicines are off-patent, some are price-controlled (at least in most of the world), some are bought at "negotiated" prices where one of the parties negotiating (the government) has the power to expropriate the patent from the producer. In other words, their prices are usually set at variable cost plus a small markup.
Hey, says Reggie the regulator, they're making a profit on each pill, so they should produce it anyway.
(Did you spot the error?)
(Wait for it...)
(Got it yet?)
Dear Reggie: pills are made in these things called "laboratories," that are really factories. Factories, you may be interested to know, have something called "capacity constraints," which means that using a production line for making one type of pill precludes that production line from making a different kind of pill. Manufacturers are in luck, though, because most production lines can be repurposed from one medication to another with relatively small configuration cost.
Companies make their decisions based on opportunity costs, not just variable costs. If they have a margin of say 90 cents/pill for growing longer eyelashes (I'm not kidding, there's a "medication" for that) and say 5 cents/pill to cure TB, they are going to dedicate as much of their production capacity to the eyelash-elongating "medication" as they can.* (They won't stop making the TB medication altogether because that would be bad for public relations.)
Funny how these things work, huh?
-----------
* Unless they can make more than eighteen times more TB pills than eyelash "medicine" pills with the same production facilities, of course.
Labels:
business,
healthcare,
management,
pharma
Tuesday, October 4, 2011
Books on teaching and presentations
During a decluttering of my place, I had to make decisions about which books to keep; these are some that I found useful for teaching and presentations, and I'm therefore keeping:
They are stacked by book size (for stability), but I'll group them in four major topics: general presentation planning and design; teaching; speechwriting; and visuals design.
1. Presentation planning and design
Edward Tufte's Beautiful Evidence is not just about making presentations, rather it's about analyzing, presenting, and consuming evidence.
Lani Arredondo's How to Present Like a Pro is the only "general presentation" book I'm keeping (and I'm still pondering that, as most of what it says is captured in my 3500-word post on preparing presentations). It's not especially good (or bad), it's just the best of the "general presentation" books I have, and there's no need for more than one. Whether I need one given Beautiful Evidence is an open question.
Donald Norman's Living With Complexity and Things That Make Us Smart are not about presentations, rather about designing cognitive artifacts (of which presentations and teaching exercises are examples) for handling complex and new units of knowledge.
Chip and Dan Heath's Made to Stick is a good book on memorability; inasmuch as we expect our students and audiences to take something away from a speech, class, or exec-ed, making memorable cognitive artifacts is an important skill to have.
Steve Krug's Don't Make Me Think is about making the process of interactions with cognitive artifacts as simple as possible (the book is mostly about the web, but the principles therein apply to presentation design as well).
Alan Cooper's The Inmates Are Running The Asylum is similar to Living With Complexity, with the added benefit of explicitly addressing the use of personas for designing complex products (a very useful product design tool for classes, I think).
I had other books on the general topic of presentations that I am donating/recycling. Most of them spend a lot of space discussing the management of stage fright, a problem with which I am not afflicted.
If I had to pick just one to keep, I'd choose Beautiful Evidence. (The others, except How To Present Like a Pro, are research-related, so I'd keep them anyway.)
2. Teaching
As I've mentioned previously, preparing instruction is different from preparing presentations. The two books I recommended then are the two books I'm keeping:
Tools for teaching, by Barbara Gross Davis covers every element of course design, class design, class management, and evaluation. It is rather focussed on institutional learning (like university courses), but many of the issues, techniques, and checklists are applicable in other instruction environments.
Designing effective instruction, by Gary Morrison, Steven Ross, and Jerrold Kemp, complements Tools for teaching. While Tools for Teaching has the underlying model of a course, this book tackles the issues of training and instruction from a professional service point of view. (In short: TfT is geared towards university classes, DEI is geared towards firm-specific Exec-Ed.)
I had other books on the general topic of teaching (and a number of books on academic life) that I am donating/recycling.
3. Speechwriting and public speaking
Speak like Churchill, stand like Lincoln, by James Humes, should be mandatory reading for anyone who ever has to make a public speech. Of any kind. Humes is a speechwriter and public speaker by profession and his book gives out practical advice on both the writing and the delivery. I have read many books on public speaking and this one is in a class of its own.
I have a few books from the Toastmasters series; I'm keeping (for now at least) Writing Great Speeches and Choosing Powerful Words, though their content overlaps a lot with Virginia Tufte's Beautiful Sentences, a book I'm definitely keeping as part of my writing set.
I'm probably keeping Richard Dowis's The Lost Art of The Great Speech as a good reference for styles and as motivation reading. (Every so often one needs to be reminded of why one does these things.)
I have other books on writing, in general, but the ones in the pile above are specific to speechwriting. I'm throwing out a few books on the business of speechwriting; they are so bad that I thought of keeping them as satire. Donating them would be an act of cruelty towards the recipients.
If I had to pick just one book on speechwriting, I'd go with Speak like Churchill, Stand like Lincoln. Hands down the best in the category, and I've read many.
4. Visuals design
Yes, the design of visuals for presentations or teaching, not Visual Design the discipline.
Edward Tufte's books are the alpha and the omega in this category. Anyone with any interest in information design should read these books carefully and reread them often.
The Non-Designer Design Book, by Robin Williams lets us in on the secrets behind what works visually and what doesn't. It really makes one appreciate the importance of what appears at first to be over-fussy unimportant details. I complement this with The Non-Designer Type Book and Robin Williams Design Workshop, the first specifically for type, the second as an elaboration of the Non-Designer Design Book.
Universal principles of design, by William Lidwell, Kristina Holden, and Jill Butler is a quick reference for design issues. I also like to peruse it regularly to get some reminders of design principles. It's organized alphabetically and each principle has a page or two, with examples.
Perhaps I'm a bit focussed on typography (a common symptom of reading design books, I'm told), but Robert Bringhurst's The Elements of Typographic Style is a really good and deeply interesting book on the subject. Much more technical than The Non-Designer Type Book, obviously, and the reason why I hesitate to switch from Adobe CS to iWork for my handouts.
Zakia and Page's Photographic Composition: A visual guide is very useful as a guide to laying out materials for impact. Designing the visual flow of a slide (or a handout) -- when there are options, of course, this is not about "reshaping" statistical charts -- helps tell a story even without narration or animation.
I had some other books on the general topic of slide design, which I am donating. I also have a collection of books on art, photography, and design in general, which affords me a reference library. (That collection I'm keeping.)
If I had to pare down the set further, the last ones I'd give up are the four Tufte books. If forced to pick just one (in addition to Beautiful Evidence, which fills the presentation category above), I'd choose The Visual Display of Quantitative Information, because that's the most germane to the material I cover.
CODA: A smaller set
Not that I'm getting rid of the books in the larger set above (that's the set that I'm keeping), but I think there's a core set of books I should reread at least once a year. Unsurprisingly, those are the same books I'd pick if I really could have only one per category (or one set for the last category):
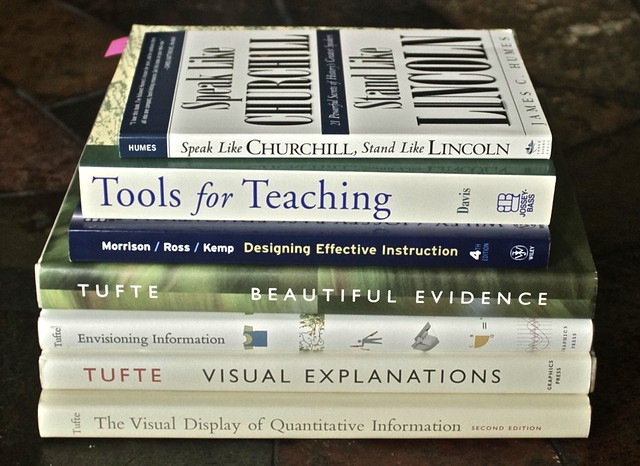
They are stacked by book size (for stability), but I'll group them in four major topics: general presentation planning and design; teaching; speechwriting; and visuals design.
1. Presentation planning and design
Edward Tufte's Beautiful Evidence is not just about making presentations, rather it's about analyzing, presenting, and consuming evidence.
Lani Arredondo's How to Present Like a Pro is the only "general presentation" book I'm keeping (and I'm still pondering that, as most of what it says is captured in my 3500-word post on preparing presentations). It's not especially good (or bad), it's just the best of the "general presentation" books I have, and there's no need for more than one. Whether I need one given Beautiful Evidence is an open question.
Donald Norman's Living With Complexity and Things That Make Us Smart are not about presentations, rather about designing cognitive artifacts (of which presentations and teaching exercises are examples) for handling complex and new units of knowledge.
Chip and Dan Heath's Made to Stick is a good book on memorability; inasmuch as we expect our students and audiences to take something away from a speech, class, or exec-ed, making memorable cognitive artifacts is an important skill to have.
Steve Krug's Don't Make Me Think is about making the process of interactions with cognitive artifacts as simple as possible (the book is mostly about the web, but the principles therein apply to presentation design as well).
Alan Cooper's The Inmates Are Running The Asylum is similar to Living With Complexity, with the added benefit of explicitly addressing the use of personas for designing complex products (a very useful product design tool for classes, I think).
I had other books on the general topic of presentations that I am donating/recycling. Most of them spend a lot of space discussing the management of stage fright, a problem with which I am not afflicted.
If I had to pick just one to keep, I'd choose Beautiful Evidence. (The others, except How To Present Like a Pro, are research-related, so I'd keep them anyway.)
2. Teaching
As I've mentioned previously, preparing instruction is different from preparing presentations. The two books I recommended then are the two books I'm keeping:
Tools for teaching, by Barbara Gross Davis covers every element of course design, class design, class management, and evaluation. It is rather focussed on institutional learning (like university courses), but many of the issues, techniques, and checklists are applicable in other instruction environments.
Designing effective instruction, by Gary Morrison, Steven Ross, and Jerrold Kemp, complements Tools for teaching. While Tools for Teaching has the underlying model of a course, this book tackles the issues of training and instruction from a professional service point of view. (In short: TfT is geared towards university classes, DEI is geared towards firm-specific Exec-Ed.)
I had other books on the general topic of teaching (and a number of books on academic life) that I am donating/recycling.
3. Speechwriting and public speaking
Speak like Churchill, stand like Lincoln, by James Humes, should be mandatory reading for anyone who ever has to make a public speech. Of any kind. Humes is a speechwriter and public speaker by profession and his book gives out practical advice on both the writing and the delivery. I have read many books on public speaking and this one is in a class of its own.
I have a few books from the Toastmasters series; I'm keeping (for now at least) Writing Great Speeches and Choosing Powerful Words, though their content overlaps a lot with Virginia Tufte's Beautiful Sentences, a book I'm definitely keeping as part of my writing set.
I'm probably keeping Richard Dowis's The Lost Art of The Great Speech as a good reference for styles and as motivation reading. (Every so often one needs to be reminded of why one does these things.)
I have other books on writing, in general, but the ones in the pile above are specific to speechwriting. I'm throwing out a few books on the business of speechwriting; they are so bad that I thought of keeping them as satire. Donating them would be an act of cruelty towards the recipients.
If I had to pick just one book on speechwriting, I'd go with Speak like Churchill, Stand like Lincoln. Hands down the best in the category, and I've read many.
4. Visuals design
Yes, the design of visuals for presentations or teaching, not Visual Design the discipline.
Edward Tufte's books are the alpha and the omega in this category. Anyone with any interest in information design should read these books carefully and reread them often.
The Non-Designer Design Book, by Robin Williams lets us in on the secrets behind what works visually and what doesn't. It really makes one appreciate the importance of what appears at first to be over-fussy unimportant details. I complement this with The Non-Designer Type Book and Robin Williams Design Workshop, the first specifically for type, the second as an elaboration of the Non-Designer Design Book.
Universal principles of design, by William Lidwell, Kristina Holden, and Jill Butler is a quick reference for design issues. I also like to peruse it regularly to get some reminders of design principles. It's organized alphabetically and each principle has a page or two, with examples.
Perhaps I'm a bit focussed on typography (a common symptom of reading design books, I'm told), but Robert Bringhurst's The Elements of Typographic Style is a really good and deeply interesting book on the subject. Much more technical than The Non-Designer Type Book, obviously, and the reason why I hesitate to switch from Adobe CS to iWork for my handouts.
Zakia and Page's Photographic Composition: A visual guide is very useful as a guide to laying out materials for impact. Designing the visual flow of a slide (or a handout) -- when there are options, of course, this is not about "reshaping" statistical charts -- helps tell a story even without narration or animation.
I had some other books on the general topic of slide design, which I am donating. I also have a collection of books on art, photography, and design in general, which affords me a reference library. (That collection I'm keeping.)
If I had to pare down the set further, the last ones I'd give up are the four Tufte books. If forced to pick just one (in addition to Beautiful Evidence, which fills the presentation category above), I'd choose The Visual Display of Quantitative Information, because that's the most germane to the material I cover.
CODA: A smaller set
Not that I'm getting rid of the books in the larger set above (that's the set that I'm keeping), but I think there's a core set of books I should reread at least once a year. Unsurprisingly, those are the same books I'd pick if I really could have only one per category (or one set for the last category):
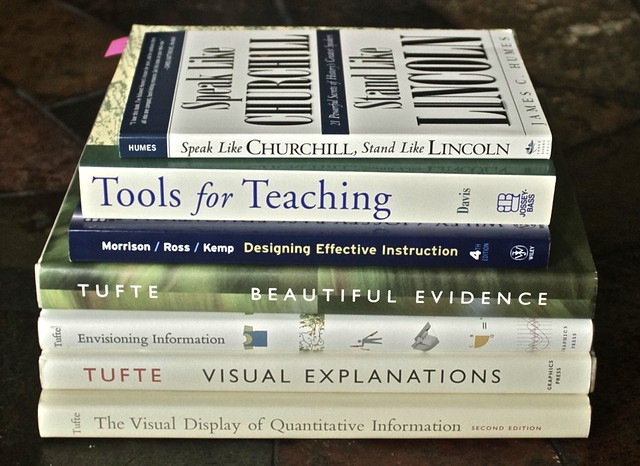
Note that the Norman, Heath Bros, Krug, Cooper books and my collection of art, photography, and design books are exempted from this choice, as they fall into separate categories: research-related or art. I also have several books on writing (some of them here).
And the books that didn't make the pile at the beginning of the post? Those, which I'm donating or recycling, make up a much larger pile (about 50% larger: 31 books on their way out).
Somewhat related posts:
Posts on presentations in my personal blog.
Posts on teaching in my personal blog.
Posts on presentations in this blog.
My 3500-word post on preparing presentations.
And the books that didn't make the pile at the beginning of the post? Those, which I'm donating or recycling, make up a much larger pile (about 50% larger: 31 books on their way out).
Somewhat related posts:
Posts on presentations in my personal blog.
Posts on teaching in my personal blog.
Posts on presentations in this blog.
My 3500-word post on preparing presentations.
Labels:
presentations,
Speechwriting,
teaching
Wednesday, September 28, 2011
What to do about psychological biases? The answer tells a lot... about you.
There are many documented cases of behavior deviating from the normative "rational" prescription of decision sciences and economics. For example, in the book Predictably Irrational, Dan Ariely tells us how he got a large number of Sloan School MBA students to change their choices using an irrelevant alternative.
The Ariely example has two groups of students choose a subscription type for The Economist. The first group was given three options to choose from: (online only, $\$60$); (paper only, $\$120$); or (paper+online, $\$120$). Overwhelmingly they chose the last option. The second group was given two options : (online only, $\$60$) or (paper+online $\$120$). Overwhelmingly they chose the first option.
Since no one chooses the (paper only, $\$120$) option, it should be irrelevant to the choices. However, removing it makes a large number of respondents change their minds. This is what is called a behavioral bias: an actual behavior that deviates from "rational" choice. (Technically these choices violate the Strong Axiom of Revealed Preference.)
(If you're not convinced that the behavior described is irrational, consider the following isomorphic problem: a waiter offers a group of people three desserts: ice cream, chocolate mousse, and fruit salad; most people choose the fruit salad, no one chooses the mousse. Then the waiter apologizes: it turns out there's no mousse. At that point most of the people who had ordered fruit salad switch to ice cream. This behavior is the same -- use some letters to represent options to remove any doubt -- as the one in Ariely's example. And few people would consider the fruit salad to ice-cream switchers rational.)
Ok, so people do, in some cases (perhaps in a majority of cases) behave in "irrational" ways, as described by the decision science and economics models. This is not entirely surprising, as those models are abstractions of idealized behavior and people are concrete physical entities with limitations and -- some argue -- faulty software.
What is really enlightening is how people who know about this feel about the biases.
IGNORE. Many academic economists and others who use economics models try to ignore these biases. Inasmuch as these biases can be more or less important depending on the decision, the persons involved, and the context, this ignorance might work for the economists, for a while. However, pretending that reality is not real is not a good foundation for Science, or even life.
ATTACK. A number of people use the existence of biases as an attack on established economics. This is how science evolves, with theories being challenged by evidence and eventually changing to incorporate the new phenomena. Some people, however, may be motivated by personal animosity towards economics and decision sciences; this creates a bad environment for knowledge evolution -- it becomes a political game, never good news for Science.
EXPLOIT. Books like Nudge make this explicit, but many people think of these biases as a way to manipulate others' behavior. Manipulate is the appropriate verb here, since these people (maybe with what they think is the best of intentions -- I understand these pave the way to someplace...) want to change others' behavior without actually telling these others what they are doing. In addition to the underhandedness that, were this a commercial application, the Nudgers would be trying to outlaw, this type of attitude reeks of "I know better than others, but they are too stupid to agree." Underhanded manipulation presented as a virtue; the world certainly has changed a lot.
ADDRESS AND MANAGE. A more productive attitude is to design decisions and information systems to minimize the effect of these biases. For example, in the decision above, both scenarios could be presented, the inconsistency pointed out, and then a separate part-worth decision could be addressed (i.e. what are each of the two elements -- print and online -- worth separately?). Note that this is the one attitude that treats behavioral biases as damage and finds way to route decisions around them, unlike the other three attitudes.
In case it's not obvious, my attitude towards these biases is to address and manage them.
The Ariely example has two groups of students choose a subscription type for The Economist. The first group was given three options to choose from: (online only, $\$60$); (paper only, $\$120$); or (paper+online, $\$120$). Overwhelmingly they chose the last option. The second group was given two options : (online only, $\$60$) or (paper+online $\$120$). Overwhelmingly they chose the first option.
Since no one chooses the (paper only, $\$120$) option, it should be irrelevant to the choices. However, removing it makes a large number of respondents change their minds. This is what is called a behavioral bias: an actual behavior that deviates from "rational" choice. (Technically these choices violate the Strong Axiom of Revealed Preference.)
(If you're not convinced that the behavior described is irrational, consider the following isomorphic problem: a waiter offers a group of people three desserts: ice cream, chocolate mousse, and fruit salad; most people choose the fruit salad, no one chooses the mousse. Then the waiter apologizes: it turns out there's no mousse. At that point most of the people who had ordered fruit salad switch to ice cream. This behavior is the same -- use some letters to represent options to remove any doubt -- as the one in Ariely's example. And few people would consider the fruit salad to ice-cream switchers rational.)
Ok, so people do, in some cases (perhaps in a majority of cases) behave in "irrational" ways, as described by the decision science and economics models. This is not entirely surprising, as those models are abstractions of idealized behavior and people are concrete physical entities with limitations and -- some argue -- faulty software.
What is really enlightening is how people who know about this feel about the biases.
IGNORE. Many academic economists and others who use economics models try to ignore these biases. Inasmuch as these biases can be more or less important depending on the decision, the persons involved, and the context, this ignorance might work for the economists, for a while. However, pretending that reality is not real is not a good foundation for Science, or even life.
ATTACK. A number of people use the existence of biases as an attack on established economics. This is how science evolves, with theories being challenged by evidence and eventually changing to incorporate the new phenomena. Some people, however, may be motivated by personal animosity towards economics and decision sciences; this creates a bad environment for knowledge evolution -- it becomes a political game, never good news for Science.
EXPLOIT. Books like Nudge make this explicit, but many people think of these biases as a way to manipulate others' behavior. Manipulate is the appropriate verb here, since these people (maybe with what they think is the best of intentions -- I understand these pave the way to someplace...) want to change others' behavior without actually telling these others what they are doing. In addition to the underhandedness that, were this a commercial application, the Nudgers would be trying to outlaw, this type of attitude reeks of "I know better than others, but they are too stupid to agree." Underhanded manipulation presented as a virtue; the world certainly has changed a lot.
ADDRESS AND MANAGE. A more productive attitude is to design decisions and information systems to minimize the effect of these biases. For example, in the decision above, both scenarios could be presented, the inconsistency pointed out, and then a separate part-worth decision could be addressed (i.e. what are each of the two elements -- print and online -- worth separately?). Note that this is the one attitude that treats behavioral biases as damage and finds way to route decisions around them, unlike the other three attitudes.
In case it's not obvious, my attitude towards these biases is to address and manage them.
Labels:
Biases,
Decision-Science,
economics,
psychology
Sunday, September 18, 2011
Probability interlude: from discrete events to continuous time
Lunchtime fun: the relationship between Bernoulli and Exponential distributions.
Let's say the probability of Joe getting a coupon for Pepsi in any given time interval $\Delta t$, say a month, is given by $p$. This probability depends on a number of things, such as intensity of couponing activity, quality of targeting, Joe not throwing away all junk mail, etc.
For a given integer number of months, $n$, we can easily compute the probability, $P$, of Joe getting at least one coupon during the period, which we'll call $t$, as
$P(n) = 1 - (1-p)^n$.
Since the period $t$ is $t= n \times \Delta t$, we can write that as
$P(t) = 1 - (1-p)^{\frac{t}{\Delta t}}.$
Or, with a bunch of assumptions that we'll assume away,
$P(t) = 1- \exp\left(t \times \frac{\log (1-p)}{\Delta t}\right).$
Note that $\log (1-p)<0$. Defining $r = - \log (1-p) /\Delta t$, we get
$P(t) = 1 - \exp (- r t)$.
And that is the relationship between the Bernoulli distribution and the Exponential distribution.
We can now build continuous-time analyses of couponing activity. Continuous analysis is much easier to do than discrete analysis. Also, though most simulators are, by computational necessity, discrete, building them based on continuous time models is usually simpler and easier to explain to managers using them.
Let's say the probability of Joe getting a coupon for Pepsi in any given time interval $\Delta t$, say a month, is given by $p$. This probability depends on a number of things, such as intensity of couponing activity, quality of targeting, Joe not throwing away all junk mail, etc.
For a given integer number of months, $n$, we can easily compute the probability, $P$, of Joe getting at least one coupon during the period, which we'll call $t$, as
$P(n) = 1 - (1-p)^n$.
Since the period $t$ is $t= n \times \Delta t$, we can write that as
$P(t) = 1 - (1-p)^{\frac{t}{\Delta t}}.$
Or, with a bunch of assumptions that we'll assume away,
$P(t) = 1- \exp\left(t \times \frac{\log (1-p)}{\Delta t}\right).$
Note that $\log (1-p)<0$. Defining $r = - \log (1-p) /\Delta t$, we get
$P(t) = 1 - \exp (- r t)$.
And that is the relationship between the Bernoulli distribution and the Exponential distribution.
We can now build continuous-time analyses of couponing activity. Continuous analysis is much easier to do than discrete analysis. Also, though most simulators are, by computational necessity, discrete, building them based on continuous time models is usually simpler and easier to explain to managers using them.
Labels:
mathematics,
Probability
Saturday, September 17, 2011
Small probabilities, big trouble.
After a long – work-related – hiatus, I'm back to blogging with a downer: the troublesome nature of small probability estimation.
The idea for this post came from a speech by Nassim Nicholas Taleb at Penn. Though the video is a bit rambling, it contains several important points. One that is particularly interesting to me is the difficulty of estimating the probability of rare events.
For illustration, let's consider a Normally distributed random variable $P$, and see what happens when small model errors are introduced. In particular we want to how the probability density $f_{P}(\cdot)$ predicted by four different models changes as a function of distance to zero, $x$. The higher the $x$ the more infrequently the event $P = x$ happens.
The densities are computed in the following table (click for larger):
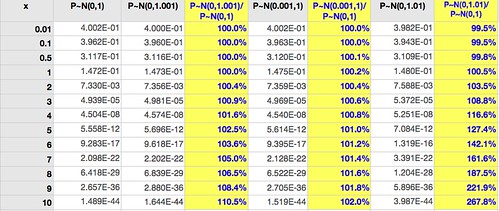
The first column gives $f_{P}(x)$ for $P \sim \mathcal{N}(0,1)$, the base case. The next column is similar except that there's a 0.1% increase in the variance (10 basis points*). The third column is the ratio of these densities. (These are not probabilities, since $P$ is a continuous variable.)
Two observations jump at us:
1. Near the mean, where most events happen, it's very difficult to separate the two cases: the ratio of the densities up to two standard deviations ($x=2$) is very close to 1.
2. Away from the mean, where events are infrequent (but potentially with high impact), the small error of 10 basis points is multiplied: at highly infrequent events ($x>7$) the density is off by over 500 basis points.
So: it's very difficult to tell the models apart with most data, but they make very different predictions for uncommon events. If these events are important when they happen, say a stock market crash, this means trouble.
Moving on, the fourth column uses $P \sim \mathcal{N}(0.001,1)$, the same 10 basis points error, but in the mean rather than the variance. Column five is the ratio of these densities to the base case.
Comparing column five with column three we see that similarly sized errors in mean estimation have less impact than errors in variance estimation. Unfortunately variance is harder to estimate accurately than the mean (it uses the mean estimate as an input, for one), so this only tells us that problems are likely to happen where they are more damaging to model predictive abilities.
Column six shows the effect of a larger variance (100 basis points off the standard, instead of 10); column seven shows the ratio of this density to the base case.
With an error of 1% in the estimate of the variance it's still hard to separate the models within two standard deviations (for a Normal distribution about 95% of all events fall within two standard deviations of the mean), but the error in density estimates at $x=7$ is 62%.
Small probability events are very hard to predict because most of the times all the information available is not enough to choose between models that have very close parameters but these models predict very different things for infrequent cases.
Told you it was a downer.
-- -- --
* Some time ago I read a criticism of this nomenclature by someone who couldn't see its purpose. The purpose is good communication design: when there's a lot of 0.01% and 0.1% being spoken in a noisy environment it's a good idea to say "one basis point" or "ten basis points" instead of "point zero one" or "zero point zero one" or "point zero zero one." It's the same reason we say "Foxtrot Universe Bravo Alpha Romeo" instead of "eff u bee a arr" in audio communication.
NOTE for probabilists appalled at my use of $P$ in $f_{P}(x)$ instead of more traditional nomenclature $f_{X}(x)$ where the uppercase $X$ would mean the variable and the lowercase $x$ the value: most people get confused when they see something like $p=\Pr(x=X)$.
The idea for this post came from a speech by Nassim Nicholas Taleb at Penn. Though the video is a bit rambling, it contains several important points. One that is particularly interesting to me is the difficulty of estimating the probability of rare events.
For illustration, let's consider a Normally distributed random variable $P$, and see what happens when small model errors are introduced. In particular we want to how the probability density $f_{P}(\cdot)$ predicted by four different models changes as a function of distance to zero, $x$. The higher the $x$ the more infrequently the event $P = x$ happens.
The densities are computed in the following table (click for larger):
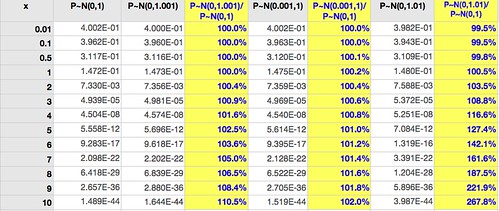
The first column gives $f_{P}(x)$ for $P \sim \mathcal{N}(0,1)$, the base case. The next column is similar except that there's a 0.1% increase in the variance (10 basis points*). The third column is the ratio of these densities. (These are not probabilities, since $P$ is a continuous variable.)
Two observations jump at us:
1. Near the mean, where most events happen, it's very difficult to separate the two cases: the ratio of the densities up to two standard deviations ($x=2$) is very close to 1.
2. Away from the mean, where events are infrequent (but potentially with high impact), the small error of 10 basis points is multiplied: at highly infrequent events ($x>7$) the density is off by over 500 basis points.
So: it's very difficult to tell the models apart with most data, but they make very different predictions for uncommon events. If these events are important when they happen, say a stock market crash, this means trouble.
Moving on, the fourth column uses $P \sim \mathcal{N}(0.001,1)$, the same 10 basis points error, but in the mean rather than the variance. Column five is the ratio of these densities to the base case.
Comparing column five with column three we see that similarly sized errors in mean estimation have less impact than errors in variance estimation. Unfortunately variance is harder to estimate accurately than the mean (it uses the mean estimate as an input, for one), so this only tells us that problems are likely to happen where they are more damaging to model predictive abilities.
Column six shows the effect of a larger variance (100 basis points off the standard, instead of 10); column seven shows the ratio of this density to the base case.
With an error of 1% in the estimate of the variance it's still hard to separate the models within two standard deviations (for a Normal distribution about 95% of all events fall within two standard deviations of the mean), but the error in density estimates at $x=7$ is 62%.
Small probability events are very hard to predict because most of the times all the information available is not enough to choose between models that have very close parameters but these models predict very different things for infrequent cases.
Told you it was a downer.
-- -- --
* Some time ago I read a criticism of this nomenclature by someone who couldn't see its purpose. The purpose is good communication design: when there's a lot of 0.01% and 0.1% being spoken in a noisy environment it's a good idea to say "one basis point" or "ten basis points" instead of "point zero one" or "zero point zero one" or "point zero zero one." It's the same reason we say "Foxtrot Universe Bravo Alpha Romeo" instead of "eff u bee a arr" in audio communication.
NOTE for probabilists appalled at my use of $P$ in $f_{P}(x)$ instead of more traditional nomenclature $f_{X}(x)$ where the uppercase $X$ would mean the variable and the lowercase $x$ the value: most people get confused when they see something like $p=\Pr(x=X)$.
Monday, August 29, 2011
Decline and fall of Western Manufacturing - a pessimistic reading of Pisano and Shih (2009)
Those who don't know history are condemned to repeat it.
Unfortunately those of us who do know history get dragged right along with the others, because we live in a world where everything is connected to everything else.
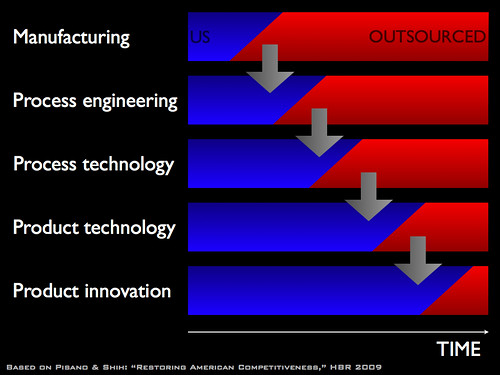
Above is my visualization of Pisano and Shih's 2009 Harvard Business Review article "Restoring American Competitiveness." This is a stylized version of a story that has happened in several industries.
Step 1: Companies start outsourcing their manufacturing operations to companies (or countries) which can perform them in a more cost-effective manner. Perhaps these companies/countries have cheaper labor, fewer costly regulations, or less overhead.
Step 2: Isolated from their manufacture, companies lose the skills for process engineering. After all, improving manufacturing processes is a task that depends on continuous experimentation and feedback from the manufacturing process. If the manufacturing process is outsourced, the necessary interaction between manufacturing and process engineers happens progressively more inside the contractor, not the original manufacturer.
Step 3: Without process engineering to motivate it, the original manufacturer (and the companies supporting it in the original country, in the diagram the US) stops investing in process technology development. For example, the companies that developed machine tools for US manufacturers in conjunction with US process engineers now have to so do with Taiwanese engineers in Taiwan, which leads to relocation of these companies and eventually of the skilled professionals.
Step 4: Because of spillovers in technological development between process technologies and product technologies (including the development of an engineering class and engineering support infrastructure), more and more product technology development is outsourced. For example, as fewer engineering jobs are available in the original country, fewer people go to engineering school; the opposite happens in the outsourced-to country, where an engineering class grows. That growth is a spillover that is seldom accounted for.
Step 5: As more and more technology development happens in the outsourced-to country, it captures more and more of the product innovation process, eventually substituting for the innovators in the original manufacturer's country. Part of this innovation may still be under contract with the original manufacturer, but the development of innovation skills in the outsourced-to country means that at some point it will have its own independent manufacturers (who will compete with the original manufacturer).
Pisano and Shih are optimists, as their article proposes solutions to slow, stop, and reverse this process of technological decline of the West (in their case, the US). It's worth a read (it's not free but it's cheaper than a day worth of lattes, m'kay?) and ends in an upbeat note.
I'm less optimistic than Pisano and Shih. Behold:
Problem 1: Too many people and too much effort dedicated to non-wealth-creating activities and too many people and too much effort aimed at stopping wealth-creating activities.
Problem 2: Lack of emphasis in useful skills (particularly STEM, entrepreneurship, and "maker" culture) in education. Sadly accompanied by a sense of entitlement and self-confidence which is inversely proportional to the actual skills.
Problem 3: Too much public discourse (politicians of both parties, news media, entertainment) which vilifies the creation of wealth and applauds the forcible redistribution of whatever wealth is created.
Problem 4: A generalized confusion between wealth and pieces of fancy green paper with pictures of dead presidents (or Ben Franklin) on them.
Problem 5: A lack of priorities or perspective beyond the immediate sectorial interests.
We are doomed!
Unfortunately those of us who do know history get dragged right along with the others, because we live in a world where everything is connected to everything else.
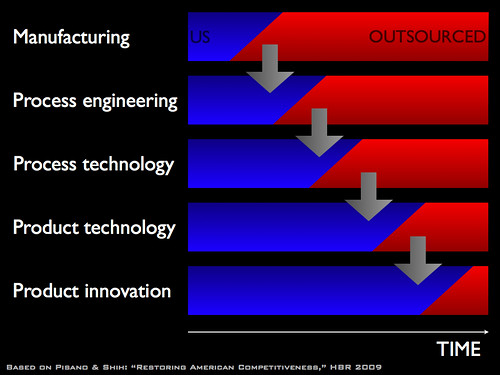
Above is my visualization of Pisano and Shih's 2009 Harvard Business Review article "Restoring American Competitiveness." This is a stylized version of a story that has happened in several industries.
Step 1: Companies start outsourcing their manufacturing operations to companies (or countries) which can perform them in a more cost-effective manner. Perhaps these companies/countries have cheaper labor, fewer costly regulations, or less overhead.
Step 2: Isolated from their manufacture, companies lose the skills for process engineering. After all, improving manufacturing processes is a task that depends on continuous experimentation and feedback from the manufacturing process. If the manufacturing process is outsourced, the necessary interaction between manufacturing and process engineers happens progressively more inside the contractor, not the original manufacturer.
Step 3: Without process engineering to motivate it, the original manufacturer (and the companies supporting it in the original country, in the diagram the US) stops investing in process technology development. For example, the companies that developed machine tools for US manufacturers in conjunction with US process engineers now have to so do with Taiwanese engineers in Taiwan, which leads to relocation of these companies and eventually of the skilled professionals.
Step 4: Because of spillovers in technological development between process technologies and product technologies (including the development of an engineering class and engineering support infrastructure), more and more product technology development is outsourced. For example, as fewer engineering jobs are available in the original country, fewer people go to engineering school; the opposite happens in the outsourced-to country, where an engineering class grows. That growth is a spillover that is seldom accounted for.
Step 5: As more and more technology development happens in the outsourced-to country, it captures more and more of the product innovation process, eventually substituting for the innovators in the original manufacturer's country. Part of this innovation may still be under contract with the original manufacturer, but the development of innovation skills in the outsourced-to country means that at some point it will have its own independent manufacturers (who will compete with the original manufacturer).
Pisano and Shih are optimists, as their article proposes solutions to slow, stop, and reverse this process of technological decline of the West (in their case, the US). It's worth a read (it's not free but it's cheaper than a day worth of lattes, m'kay?) and ends in an upbeat note.
I'm less optimistic than Pisano and Shih. Behold:
Problem 1: Too many people and too much effort dedicated to non-wealth-creating activities and too many people and too much effort aimed at stopping wealth-creating activities.
Problem 2: Lack of emphasis in useful skills (particularly STEM, entrepreneurship, and "maker" culture) in education. Sadly accompanied by a sense of entitlement and self-confidence which is inversely proportional to the actual skills.
Problem 3: Too much public discourse (politicians of both parties, news media, entertainment) which vilifies the creation of wealth and applauds the forcible redistribution of whatever wealth is created.
Problem 4: A generalized confusion between wealth and pieces of fancy green paper with pictures of dead presidents (or Ben Franklin) on them.
Problem 5: A lack of priorities or perspective beyond the immediate sectorial interests.
We are doomed!
Labels:
business,
management,
manufacturing,
Strategy
Monday, August 22, 2011
Preparing instruction is different from preparing presentations
The title bears repeating, as many people confuse instruction and presentation preparation skills and criteria for success: Preparing instruction is different from preparing presentations.
My 3500-word post on preparing presentations is exactly for that purpose, preparing presentations. I could try to write a post for preparing instruction, but it would quickly get to book size. In fact, I recommend several books in this post describing the evolution of information design in my teaching approach. (The most relevant books for teaching are at the addendum to this post.)
I made a diagram depicting my process of preparing for a instruction event (the diagram was for my personal use, but there's no reason not to share it; click for larger):
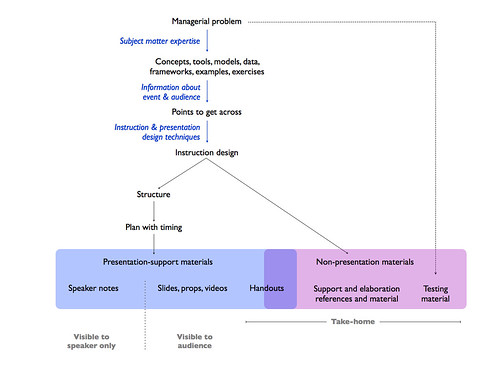
And, for comparison, the process for preparing presentations:
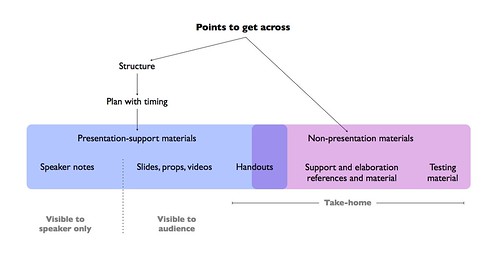
Because they look similar, I need to point out that the tools used in each phase of the process are different for presentations and for instruction.
I'm a big fan of participant-centered learning (though not necessarily the HBS cases that people always associate with PCL); the idea is simple: students learn from doing, not from watching the instructor do. So, many of the "materials" (more precisely, most of the time in the "plan with timing" part of the diagram) in an instruction event are audience work: discussions, examples brought by the audience (to complement those brought by the instructor) and exercises. These are not materials that can be used in a speech or a presentation to a large audience.
Also, while a story works as a motivator for both presentations and instruction, I tend to use exercises or problems as motivators for instruction. For example, I start a class on promotion metrics by asking "how do you measure the lift" of some promotional activity, and proceed from there. By making it a management task that they have to do as part of their jobs, I get some extra attention from the audience. Plus, they can immediately see how the class will help them with their jobs.*
There are presentations that are mostly for instruction purposes, and there are parts of instruction events that are presentations. But never mistake one for the other: preparing instruction is different from preparing presentations.
Though so much instruction is so poorly prepared that even the basics of presentation preparation will help make instruction less of a disaster, that's just a step towards instruction-specific preparation.
- - - - - - - - - - - -
*I have a large variety of exercises for each knowledge unit I teach, and they are not all of the form "here's a problem, what's the solution?" Some are of the forms "here's what a company is doing, what are they trying to achieve?" and "here's a problem, here's what the company is doing, what is wrong with that?"
Addendum: Two books on preparation (and delivery) of instruction, from the post describing the evolution of information design in my teaching approach:
Tools for teaching, by Barbara Gross Davis covers every element of course design, class design, class management, and evaluation. It is rather focussed on institutional learning (like university courses), but many of the issues, techniques, and checklists are applicable in other instruction environments.
Designing effective instruction, by Gary Morrison, Steven Ross, and Jerrold Kemp, complements Tools for teaching. While TfT has the underlying model of a class, this book tackles the issues of training and instruction from a professional service point of view. (In short: TfT is geared towards university classes, DEI is geared towards firm-specific Exec-Ed.)
My 3500-word post on preparing presentations is exactly for that purpose, preparing presentations. I could try to write a post for preparing instruction, but it would quickly get to book size. In fact, I recommend several books in this post describing the evolution of information design in my teaching approach. (The most relevant books for teaching are at the addendum to this post.)
I made a diagram depicting my process of preparing for a instruction event (the diagram was for my personal use, but there's no reason not to share it; click for larger):
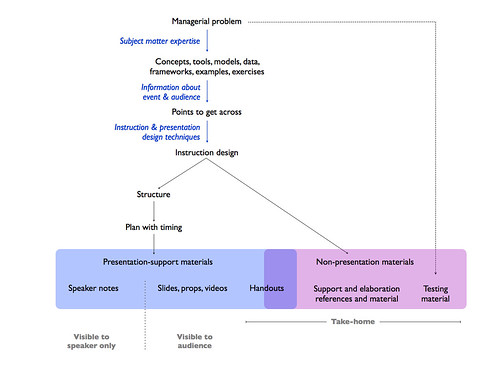
And, for comparison, the process for preparing presentations:
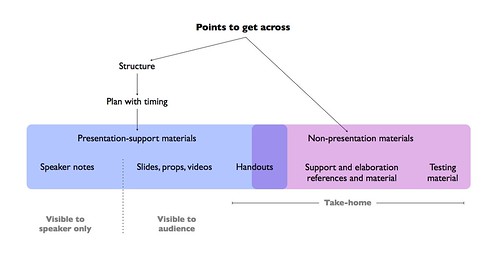
Because they look similar, I need to point out that the tools used in each phase of the process are different for presentations and for instruction.
I'm a big fan of participant-centered learning (though not necessarily the HBS cases that people always associate with PCL); the idea is simple: students learn from doing, not from watching the instructor do. So, many of the "materials" (more precisely, most of the time in the "plan with timing" part of the diagram) in an instruction event are audience work: discussions, examples brought by the audience (to complement those brought by the instructor) and exercises. These are not materials that can be used in a speech or a presentation to a large audience.
Also, while a story works as a motivator for both presentations and instruction, I tend to use exercises or problems as motivators for instruction. For example, I start a class on promotion metrics by asking "how do you measure the lift" of some promotional activity, and proceed from there. By making it a management task that they have to do as part of their jobs, I get some extra attention from the audience. Plus, they can immediately see how the class will help them with their jobs.*
There are presentations that are mostly for instruction purposes, and there are parts of instruction events that are presentations. But never mistake one for the other: preparing instruction is different from preparing presentations.
Though so much instruction is so poorly prepared that even the basics of presentation preparation will help make instruction less of a disaster, that's just a step towards instruction-specific preparation.
- - - - - - - - - - - -
*I have a large variety of exercises for each knowledge unit I teach, and they are not all of the form "here's a problem, what's the solution?" Some are of the forms "here's what a company is doing, what are they trying to achieve?" and "here's a problem, here's what the company is doing, what is wrong with that?"
Addendum: Two books on preparation (and delivery) of instruction, from the post describing the evolution of information design in my teaching approach:
Tools for teaching, by Barbara Gross Davis covers every element of course design, class design, class management, and evaluation. It is rather focussed on institutional learning (like university courses), but many of the issues, techniques, and checklists are applicable in other instruction environments.
Designing effective instruction, by Gary Morrison, Steven Ross, and Jerrold Kemp, complements Tools for teaching. While TfT has the underlying model of a class, this book tackles the issues of training and instruction from a professional service point of view. (In short: TfT is geared towards university classes, DEI is geared towards firm-specific Exec-Ed.)
Labels:
presentations,
teaching
Thursday, July 28, 2011
A simple, often overlooked, problem with models
There are just too many possibilities.
Let's say we have one dependent variable, $y$, and ten independent variables, $x_1,\ldots,x_{10}$. How many models can we build? For simplicity let's keep our formulation linear (in the usual sense of the word, that is linear in the coefficients; see footnote).
Inexcusably wrong answer: 11 models.
Wrong answer: 1024 models.
Right-ish answer: $1.8 \times 10^{308}$ models.
Right answer: an infinity of models.
Ok, 1024 is the number of models which include at most one instance of each variable and no interaction. Something like
$ y = \beta_0 + \beta_1 \, x_1 + \beta_3 \, x_3 + \beta_7 \, x_7$ ,
of which there are $2^{10}$ models. (Since the constant $\beta_0$ can be zero by calibration, we'll include it in all models -- otherwise we'd have to demean the $y$.)
Once we consider possible interactions among variables, like $x_1 x_7 x_8$ for example, a three-way interaction, there are $2^{10}$ variables and interactions and therefore $2^{2^{10}}= 1.8 \times 10^{308}$ possible models with all interactions. For comparison, the number of atoms in the known universe is estimated to be in the order of $10^{80}$.
Of course, each variable can enter the model in a variety of functional forms: $x_1^{2}$, $\log(x_7)$, $\sin(5 \, x_9)$ or $x_3^{-x_{2}/2}$, for example, making it an infinite number of possibilities. (And there can be interactions between these different functions of different variables, obviously.)
(Added on August 11th.) Using polynomial approximations for generalized functions, say to the fourth degree, the total number of interactions is now $5^{10}=9765625$, as any variable may enter an interaction in one of five orders (0 through 4), and the total number of models is $2^{5^{10}}$ or around $10^{3255000}$. (End of addition.)
So here's a combinatorial riddle for statisticians: how can you identify a model out of, let's be generous, $1.8 \times 10^{308}$ with data in the exa- or petabyte range? That's almost three hundred orders of magnitude too little, methinks.
The main point is that any non-trivial set of variables can be modeled in a vast number of ways, which means that a limited number of models presented for appreciation (or review) necessarily includes an inordinate amount of judgement from the model-builder.
It's unavoidable, but seldom acknowledged.
--------------
The "linear in coefficients" point is the following. Take the following formulation, which is clearly non-linear in the $x$:
$y = \beta_0 + \beta_1 \, x_1^{1/4} + \beta_2 \, x_1 \, x_7$
but can be made linear very easily by making two changes of variables: $ z_1 = x_1^{1/4}$ and $z_2 = x_1 \, x_7$.
In contrast, the model $y = \alpha \, \sin( \omega \, t )$ cannot be linearized in coefficients $\alpha$ and $\omega$.
Let's say we have one dependent variable, $y$, and ten independent variables, $x_1,\ldots,x_{10}$. How many models can we build? For simplicity let's keep our formulation linear (in the usual sense of the word, that is linear in the coefficients; see footnote).
Inexcusably wrong answer: 11 models.
Wrong answer: 1024 models.
Right-ish answer: $1.8 \times 10^{308}$ models.
Right answer: an infinity of models.
Ok, 1024 is the number of models which include at most one instance of each variable and no interaction. Something like
$ y = \beta_0 + \beta_1 \, x_1 + \beta_3 \, x_3 + \beta_7 \, x_7$ ,
of which there are $2^{10}$ models. (Since the constant $\beta_0$ can be zero by calibration, we'll include it in all models -- otherwise we'd have to demean the $y$.)
Once we consider possible interactions among variables, like $x_1 x_7 x_8$ for example, a three-way interaction, there are $2^{10}$ variables and interactions and therefore $2^{2^{10}}= 1.8 \times 10^{308}$ possible models with all interactions. For comparison, the number of atoms in the known universe is estimated to be in the order of $10^{80}$.
Of course, each variable can enter the model in a variety of functional forms: $x_1^{2}$, $\log(x_7)$, $\sin(5 \, x_9)$ or $x_3^{-x_{2}/2}$, for example, making it an infinite number of possibilities. (And there can be interactions between these different functions of different variables, obviously.)
(Added on August 11th.) Using polynomial approximations for generalized functions, say to the fourth degree, the total number of interactions is now $5^{10}=9765625$, as any variable may enter an interaction in one of five orders (0 through 4), and the total number of models is $2^{5^{10}}$ or around $10^{3255000}$. (End of addition.)
So here's a combinatorial riddle for statisticians: how can you identify a model out of, let's be generous, $1.8 \times 10^{308}$ with data in the exa- or petabyte range? That's almost three hundred orders of magnitude too little, methinks.
The main point is that any non-trivial set of variables can be modeled in a vast number of ways, which means that a limited number of models presented for appreciation (or review) necessarily includes an inordinate amount of judgement from the model-builder.
It's unavoidable, but seldom acknowledged.
--------------
The "linear in coefficients" point is the following. Take the following formulation, which is clearly non-linear in the $x$:
$y = \beta_0 + \beta_1 \, x_1^{1/4} + \beta_2 \, x_1 \, x_7$
but can be made linear very easily by making two changes of variables: $ z_1 = x_1^{1/4}$ and $z_2 = x_1 \, x_7$.
In contrast, the model $y = \alpha \, \sin( \omega \, t )$ cannot be linearized in coefficients $\alpha$ and $\omega$.
Labels:
analytics,
combinatorics,
model-building,
models,
technical
Sunday, July 24, 2011
Three thoughts on presentation advice
As someone who makes presentations for a living,* I regularly peruse several blogs and forums on presentations. Here are three thoughts on presentation advice, inspired by that perusal.
1. The problem with much presentation advice is that it's a meta exercise: a presentation about presentations. And it falls into what I like to call the Norman Critique of Tufte's Table Argument (NCoTTA). From Don Norman's essay "In Defense Of Powerpoint":
2. When an attendee of a short talk I recently gave asked me for quick advice on presentations I said: have a simple clear statement, in complete sentences, of what your presentation is supposed to achieve. He was flummoxed; I assume he wanted the secret sauce for my slides.
Here's a slide from that talk:
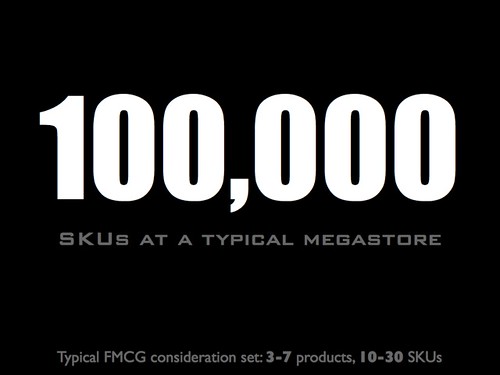
It's obvious that there is no secret sauce here; extending the cooking metaphor, what that slide shows is a good marinade: preparation. Though many presentation advice websites talk about rehearsal and working the room as preparation, what I mean is what this 3500-word post explains.
For example, knowing what the 100,000 SKU statistic is for, I chose to put the size of FMCG consideration sets as a footer, to contextualize the big number. Different uses of the big number get different footers to put it into the appropriate perspective. If all I wanted to do was illustrate how big that number is, I could say "if you bought a different SKU every day, you'd need almost 300 years to go through them all."
Most advice on presentations will not be useful because the content and the context of the presentation are much more important to the design of the presentation than generic rules. (Hence the NCoTTA problem so much advice has. Ditto for this slide, since I didn't explain what the talk was about.)
3. Speaking of Tufte, one of the things that separates him from the other presentation advocates is that he takes a full view of the communication process (partially illustrated in this post): from the speaker's data to the receiver's understanding. Here's a simple diagram to illustrate the sequence:
Most presentation advice is about the creation and, especially, the delivery of presentations. Tufte stands more or less alone as one who discusses the receiving and processing of presentation material: how to pay attention (not just being "engaged," but actually processing the information and checking for unstated assumptions, logical fallacies, psychological biases, or innumeracy) and how to elaborate on one's own, given presentation materials.
Other than Tufte and his constant reminder that receiving a presentation is an active process rather than a passive event, presentation coaches focus almost all their attention on the presenter-side processes. Many "Tufte followers" also miss this point: when processing a presentation by someone else they focus on the presentation itself (the slides, the design, the handouts) instead of the content of the presentation, i.e. the insights.
-- -- -- --
* Among other things, like teaching, creating original research, and writing.
1. The problem with much presentation advice is that it's a meta exercise: a presentation about presentations. And it falls into what I like to call the Norman Critique of Tufte's Table Argument (NCoTTA). From Don Norman's essay "In Defense Of Powerpoint":
Tufte doesn't overload the audience in his own talks—but that is because he doesn't present data as data, he presents data as examples of what slides and graphical displays might look like, so the fact that the audience might not have time to assimilate all the information is irrelevant.It's funny that Tufte is actually one of the people who least deserve the NCoTTA; most presentation coaches make that error more often and to greater depths.
2. When an attendee of a short talk I recently gave asked me for quick advice on presentations I said: have a simple clear statement, in complete sentences, of what your presentation is supposed to achieve. He was flummoxed; I assume he wanted the secret sauce for my slides.
Here's a slide from that talk:
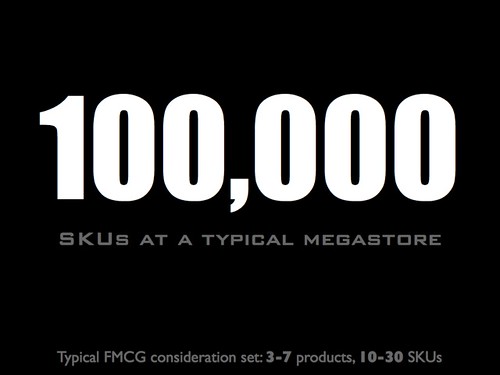
It's obvious that there is no secret sauce here; extending the cooking metaphor, what that slide shows is a good marinade: preparation. Though many presentation advice websites talk about rehearsal and working the room as preparation, what I mean is what this 3500-word post explains.
For example, knowing what the 100,000 SKU statistic is for, I chose to put the size of FMCG consideration sets as a footer, to contextualize the big number. Different uses of the big number get different footers to put it into the appropriate perspective. If all I wanted to do was illustrate how big that number is, I could say "if you bought a different SKU every day, you'd need almost 300 years to go through them all."
Most advice on presentations will not be useful because the content and the context of the presentation are much more important to the design of the presentation than generic rules. (Hence the NCoTTA problem so much advice has. Ditto for this slide, since I didn't explain what the talk was about.)
3. Speaking of Tufte, one of the things that separates him from the other presentation advocates is that he takes a full view of the communication process (partially illustrated in this post): from the speaker's data to the receiver's understanding. Here's a simple diagram to illustrate the sequence:
Most presentation advice is about the creation and, especially, the delivery of presentations. Tufte stands more or less alone as one who discusses the receiving and processing of presentation material: how to pay attention (not just being "engaged," but actually processing the information and checking for unstated assumptions, logical fallacies, psychological biases, or innumeracy) and how to elaborate on one's own, given presentation materials.
Other than Tufte and his constant reminder that receiving a presentation is an active process rather than a passive event, presentation coaches focus almost all their attention on the presenter-side processes. Many "Tufte followers" also miss this point: when processing a presentation by someone else they focus on the presentation itself (the slides, the design, the handouts) instead of the content of the presentation, i.e. the insights.
-- -- -- --
* Among other things, like teaching, creating original research, and writing.
Labels:
presentations
Friday, July 15, 2011
Adaptation, the key to success - Tim Harford at TED
This TED talk, Tim Harford on the folly of assuming one can control complex systems, is worth watching:
I have blogged about the problems with understanding causality in complex systems (in my case a system that is deceptively simple to describe but has complex behavior) before. I have Tim Harford's book Adapt, but haven't finished it yet; I will blog book notes (especially now that the Kindle App allows for copy-paste).
Experimentation, evolution, adaptation: the secret to a successful complex system. As in Nature so in business (and possibly other management fields).
I have blogged about the problems with understanding causality in complex systems (in my case a system that is deceptively simple to describe but has complex behavior) before. I have Tim Harford's book Adapt, but haven't finished it yet; I will blog book notes (especially now that the Kindle App allows for copy-paste).
Experimentation, evolution, adaptation: the secret to a successful complex system. As in Nature so in business (and possibly other management fields).
Labels:
Adaptation,
evolution,
management,
TED talk,
Tim Harford
Wednesday, July 6, 2011
Thoughts on the Kenan-Flagler $89,000 online MBA (and the MBA degree in general)
I saw the news this morning that the Kenan-Flagler Business School at the University of North Carolina—Chapel Hill is starting a new online MBA and charging the same as for its in-class MBA, $\$89,000$.
Online MBAs have so far been mostly consigned to the low end of the MBA spectrum; Kenan-Flagler is a serious school with serious quality, so this is a game-changer. Which raises the important question:
Can a online MBA be worth the same as a regular, in-class MBA?
Futurology is a field fraught with error, so let's do what smart managers do at the beginning of a category lifecycle: think carefully about the likely path of the value proposition and the revenue models. The revenue model here is tuition plus alumni donations, same as for a in-class MBA; let's analyze the four components of the value proposition:
1. Technical business material. Things like how to value a put option; how to measure consumer preferences; how to brief an advertising agency; how to organize a value chain; how to analyze the potential of a market. These technical materials are learned the same way every other technical material is (math, science, engineering): mostly by practice. Practice comes from preparing for in-class discussions or homework. So, this part of the MBA value proposition is easily transferred to a online (or even textbook-based) education.
We may like to think that students are learning the technical material in class – because we are so good at explaining it, of course – but the students only learn the material for real when they put it into practice. More often than not, when they are gearing up to solve problems or analyze cases they have to review their notes or read the textbook. All this can work in a self-guided study, be it from the textbook or from online materials. It all depends on how the motivation (aka the assessment) is executed.
2. Managerial skills. Things like leadership, decision-making on-the-fly, presentation, consensus-building, teamwork. These are essential parts of Participant-Centered Learning, and very hard to do online. There's a point in a manager's training where she has to stop analyzing, raise her hand and – in front of a group of people who are trying to find fault with it – present her view of the case. The ability to convince others and get them to execute your decisions is fundamental to the job of manager and this type of experience does require the presence in a classroom.
In fact many critics of the MBA degree as preparation for management jobs state that the in-class experience is not enough to develop these skills and should be complemented with specific soft-skill development exercises, which – needless to say – have to be done in-class.
This might sound trivial, but many students have told me that, before doing it, they had no idea what it felt like to be called upon to defend a decision that they were 51.5% sure of and make it sound convincing (otherwise it would be dead on arrival). These are the kind of skills that make the difference between a back-office analyst and a line manager.
3. Networking and the broader community. Some non-MBAs, who tend to believe in conspiracies to explain their personal shortcomings, dismiss the MBA degree as just a network-building exercise. That is incorrect, but the network one creates as part of one's MBA is a valuable part of the program. A consultant might look good "on paper," but you save your firm a lot of grief (and money) because your old Strategy teammate told you that the guy can't tell a experience effect from a sound effect. This kind of networking will only develop with continued physical proximity.
But the community doesn't end there: there are the other cohorts (older and younger) and even the contacts with faculty (which in a business school may be more useful than, say, in a humanities school). There's also the broader campus community; some MBA students at a school that shall remain nameless, located close to the Longfellow Bridge on Memorial Drive in Cambridge, MA, use their affiliation with the larger Institute to contact faculty in areas that they might find useful; engineering comes to mind. This broader community might be available to online students, especially in this age of email, instant messaging, and videoconferencing.
4. Screening and signaling. Getting accepted into the program, completing the coursework, and paying a high tuition on the expectation that future earnings will more than make up for it are all signals a student sends to the market. The more selective the school is with its incoming class, the more informative that signal is. Since the same criteria can be applied to online and in-class students, the signal should be the same. (Whether the market accepts that the criteria are the same, that's a different story.)
At first I was flabbergasted that K-F was going to charge the same for the online as for the in-class MBA, but now I think that's actually smart on two levels: one, by having the same price for both, K-F signals that both programs are in fact two variants of the same degree; two, by keeping it expensive, they feed the third component of the signal, that those enrolling expect to make a lot of money, which means work hard.
So, what is the verdict?
On one hand, there are a few serious impediments to delivering the full value proposition of an MBA through a online environment. On the other hand, some of those issues can be mitigated with short "in campus" events. And, some people point out, many MBA students never really get the "soft-skill" parts that would be missing from an online MBA; even at K-F. (Even at Halberd, come to think of it.)
The market will decide, but a-priori there's no reason why, at least for technical jobs in business (including most of the consulting and finance jobs that MBAs crave) this wouldn't be a useful program.
Online MBAs have so far been mostly consigned to the low end of the MBA spectrum; Kenan-Flagler is a serious school with serious quality, so this is a game-changer. Which raises the important question:
Can a online MBA be worth the same as a regular, in-class MBA?
Futurology is a field fraught with error, so let's do what smart managers do at the beginning of a category lifecycle: think carefully about the likely path of the value proposition and the revenue models. The revenue model here is tuition plus alumni donations, same as for a in-class MBA; let's analyze the four components of the value proposition:
1. Technical business material. Things like how to value a put option; how to measure consumer preferences; how to brief an advertising agency; how to organize a value chain; how to analyze the potential of a market. These technical materials are learned the same way every other technical material is (math, science, engineering): mostly by practice. Practice comes from preparing for in-class discussions or homework. So, this part of the MBA value proposition is easily transferred to a online (or even textbook-based) education.
We may like to think that students are learning the technical material in class – because we are so good at explaining it, of course – but the students only learn the material for real when they put it into practice. More often than not, when they are gearing up to solve problems or analyze cases they have to review their notes or read the textbook. All this can work in a self-guided study, be it from the textbook or from online materials. It all depends on how the motivation (aka the assessment) is executed.
2. Managerial skills. Things like leadership, decision-making on-the-fly, presentation, consensus-building, teamwork. These are essential parts of Participant-Centered Learning, and very hard to do online. There's a point in a manager's training where she has to stop analyzing, raise her hand and – in front of a group of people who are trying to find fault with it – present her view of the case. The ability to convince others and get them to execute your decisions is fundamental to the job of manager and this type of experience does require the presence in a classroom.
In fact many critics of the MBA degree as preparation for management jobs state that the in-class experience is not enough to develop these skills and should be complemented with specific soft-skill development exercises, which – needless to say – have to be done in-class.
This might sound trivial, but many students have told me that, before doing it, they had no idea what it felt like to be called upon to defend a decision that they were 51.5% sure of and make it sound convincing (otherwise it would be dead on arrival). These are the kind of skills that make the difference between a back-office analyst and a line manager.
3. Networking and the broader community. Some non-MBAs, who tend to believe in conspiracies to explain their personal shortcomings, dismiss the MBA degree as just a network-building exercise. That is incorrect, but the network one creates as part of one's MBA is a valuable part of the program. A consultant might look good "on paper," but you save your firm a lot of grief (and money) because your old Strategy teammate told you that the guy can't tell a experience effect from a sound effect. This kind of networking will only develop with continued physical proximity.
But the community doesn't end there: there are the other cohorts (older and younger) and even the contacts with faculty (which in a business school may be more useful than, say, in a humanities school). There's also the broader campus community; some MBA students at a school that shall remain nameless, located close to the Longfellow Bridge on Memorial Drive in Cambridge, MA, use their affiliation with the larger Institute to contact faculty in areas that they might find useful; engineering comes to mind. This broader community might be available to online students, especially in this age of email, instant messaging, and videoconferencing.
4. Screening and signaling. Getting accepted into the program, completing the coursework, and paying a high tuition on the expectation that future earnings will more than make up for it are all signals a student sends to the market. The more selective the school is with its incoming class, the more informative that signal is. Since the same criteria can be applied to online and in-class students, the signal should be the same. (Whether the market accepts that the criteria are the same, that's a different story.)
At first I was flabbergasted that K-F was going to charge the same for the online as for the in-class MBA, but now I think that's actually smart on two levels: one, by having the same price for both, K-F signals that both programs are in fact two variants of the same degree; two, by keeping it expensive, they feed the third component of the signal, that those enrolling expect to make a lot of money, which means work hard.
So, what is the verdict?
On one hand, there are a few serious impediments to delivering the full value proposition of an MBA through a online environment. On the other hand, some of those issues can be mitigated with short "in campus" events. And, some people point out, many MBA students never really get the "soft-skill" parts that would be missing from an online MBA; even at K-F. (Even at Halberd, come to think of it.)
The market will decide, but a-priori there's no reason why, at least for technical jobs in business (including most of the consulting and finance jobs that MBAs crave) this wouldn't be a useful program.
Labels:
business,
education,
management,
MBAs
Subscribe to:
Posts (Atom)