Perhaps I shouldn't try to make resolutions: I resolved to blog book notes till the end of the year, and instead I'm writing something about estimation.
A power law is a relationship of the form $y = \gamma_0 x^{\gamma_1}$ and can be linearized for estimation using OLS (with a very stretchy assumption on stochastic disturbances, but let's not quibble) into
$\log(y) = \beta_0 + \beta_1 \log(x) +\epsilon$,
from which the original parameters can be trivially recovered:
$\hat\gamma_0 = \exp(\hat\beta_0)$ and $\hat\gamma_1 = \hat\beta_1$.
Power laws are plentiful in Nature, especially when one includes the degree distribution of social networks in a – generous and uncommon, I admit it – definition of Nature. An usually proposed source of power law degree distribution is preferential attachment in network formation: the probability of a new node $i$ being connected to an old node $j$ is an increasing function of the degree of $j$.
The problem with power laws in the wild is that they are really hard to estimate precisely, and I got very annoyed at the glibness of some articles, which report estimation of power laws in highly dequantized manner: they don't actually show the estimates or their descriptive statistics, only charts with no error bars.
Here's my problem: it's well-known that even small stochastic disturbances can make parameter identification in power law data very difficult. And yet, that is never mentioned in those papers. This omission, coupled with the lack of actual estimates and their descriptive statistics, is unforgivable. And suspicious.
Perhaps this needs a couple of numerical examples to clarify; as they say at the end of each season of television shows now:
– To be continued –
Non-work posts by Jose Camoes Silva; repurposed in May 2019 as a blog mostly about innumeracy and related matters, though not exclusively.
Wednesday, December 21, 2011
Tuesday, December 20, 2011
Marginalia: Writing in one's books
I've done it for a long time now, shocking behavior though it is to some of my family and friends.
WHY I make notes
Some of my family members and friends are shocked that I write in my books. The reasons to keep the books in pristine condition vary from maintaining resale value (not an issue for me, as I don't think of books as transient presences in my life) to keeping the integrity of the author's work. Obviously, if I had a first edition of Newton's Principia, I wouldn't write on in; the books I write on are workaday copies, many of them cheap paperbacks or technical books.
The reason why I makes notes is threefold:
To better understand the book as I read it. Actively reading a book, especially a non-fiction or work book, is essentially a dialog between the book and the knowledge I can access, both in my mind and in outside references. Deciding what is important enough to highlight and what points deserve further elaboration in the form of commentary or an example that I furnish, makes reading a much more immersive experience than simply processing the words.
To collect my ideas from several readings (I read many books more than once) into a place where they are not lost. Sometimes points from a previous reading are more clarifying to me than the text itself, sometimes I disagree vehemently with what I wrote before.
To refer to later when I need to find something in the book. This is particularly important in books that I read for work, in particular for technical books where many of the details have been left out (for space reasons) but I added notes that fill those in for the parts I care about.
WHAT types of notes I make
In an earlier post about marginalia on my personal blog I included this image (click for bigger),
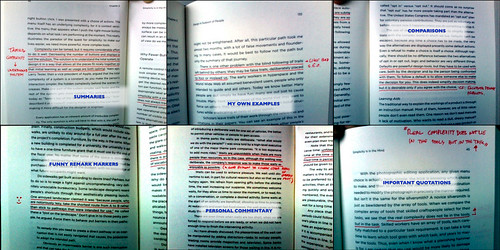
showing some notes I made while reading the book Living With Complexity, by Donald Norman. These notes fell into six cases:
Summaries of the arguments in text. Often texts will take long circuitous routes to get to the point. (Norman's book is not one of these.) I tend to write quick summaries, usually in implication form like the one above, that cut down the entropy.
My examples to complement the text. Sometimes I happen to know better examples, or examples that I prefer, than those in the book; in that case I tend to note them in the book so that the example is always connected to the context in which I thought of it. This is particularly useful in work books (and papers, of course) when I turn them into teaching or executive education materials.
Comparisons with external materials. In this case I make a note to compare Norman's point about default choices with the problems Facebook faced in similar matters regarding its privacy.
Notable passages. Marking funny passages with smiley faces and surprising passages with an exclamation point helps find these when browsing the book quickly. Occasionally I also mark passages for style or felicitous turn of phrase, typically with "nice!" on the margin.
Personal commentary. Sometimes the text provokes some reaction that I think is work recording in the book. I don't write review-like commentary in books as a general rule, but I might note something about missing or hidden assumptions, innumeracy, biases, statistical issues; I might also comment positively on an idea, for example, that I had never thought of except for the text.
Quotable passages. These are self-explanatory and particularly easy to make on eBooks. Here's one from George Orwell's Homage To Catalonia:
A few other types of marginalia that I have used in other books:
Proofs and analysis to complement what's in the text. As an example, in a PNAS paper on predictions based on search, the authors call $\log(y) = \beta_0 + \beta_1 \log(x)$ a linear model, with the logarithms used to account for the skewness of the variables. I inserted a note that this is clearly a power law relationship, not a linear relationship, with the two steps of algebra that show $y = e^{\beta_0} \times x^{\beta_1}$, in case I happen to be distracted when I reread this paper and can't think through the baby math.
Adding missing references or checking the references (which sometime are incorrect, in which case I correct them). Yep, I'm an academic nerd at heart; but these are important, like a chain of custody for evidence or the provenance records for a work of art.
Diagrams clarifying complicated points. I do this in part because I like visual thinking and in part because if I ever need to present the material to an audience I'll have a starting point for visual support design.
Data that complements the text. Sometimes the text is dequantized and refers to a story for which data is available. I find that adding the data to the story helps me get a better perspective and also if I ever want to use the story I'll have the data there to make a better case.
Counter-arguments. Sometimes I disagree with the text, or at least with the lack of feasible counter-arguments (even when I agree with a position I don't like that the author presents the opposing points of view only in strawman form), so I write the counter-arguments in order to remind me that they exist and the presentation in the text doesn't do them justice.
Markers for things that I want to get. For example, while reading Ted Gioia's The History of Jazz, I marked several recordings that he mentions for acquisition; when reading technical papers I tend to mark the references I want to check; when reading reviews I tend to add things to wishlists (though I also prune these wishlists often).
HOW to make notes
A few practical points for writing marginalia:
Highlighters are not good for long-term notes. They either darken significantly, making it hard to read the highlighted text, or they fade, losing the highlight. I prefer underlining with a high contrast color for short sentences or segments or marking beginning and end of passages on the margin.
Margins are not the only place. I add free-standing inserts, usually in the form of large Post-Its or pieces of paper. Important management tip: write the page number the note refers to on the note.
Transcribing important notes to a searchable format (a text file on my laptop) makes it easy to find stuff later. This is one of the advantages of eBooks of the various types (Kindle, iBook, O'Reilly PDFs), making it easy to search notes and highlights.
Keeping a commonplace book of felicitous turns of phrase (the ones in the books and the ones I come up with) either in a file or on an old-style paper journal helps me become a better writer.
-- -- -- --
Note: This blog may become a little more varied in topics as I decided to write posts more often to practice writing for a general audience. After all, the best way to become a better writer is to write and let others see it. (No comments on the blog, but plenty of ones by email from people I know.)
WHY I make notes
Some of my family members and friends are shocked that I write in my books. The reasons to keep the books in pristine condition vary from maintaining resale value (not an issue for me, as I don't think of books as transient presences in my life) to keeping the integrity of the author's work. Obviously, if I had a first edition of Newton's Principia, I wouldn't write on in; the books I write on are workaday copies, many of them cheap paperbacks or technical books.
The reason why I makes notes is threefold:
To better understand the book as I read it. Actively reading a book, especially a non-fiction or work book, is essentially a dialog between the book and the knowledge I can access, both in my mind and in outside references. Deciding what is important enough to highlight and what points deserve further elaboration in the form of commentary or an example that I furnish, makes reading a much more immersive experience than simply processing the words.
To collect my ideas from several readings (I read many books more than once) into a place where they are not lost. Sometimes points from a previous reading are more clarifying to me than the text itself, sometimes I disagree vehemently with what I wrote before.
To refer to later when I need to find something in the book. This is particularly important in books that I read for work, in particular for technical books where many of the details have been left out (for space reasons) but I added notes that fill those in for the parts I care about.
WHAT types of notes I make
In an earlier post about marginalia on my personal blog I included this image (click for bigger),
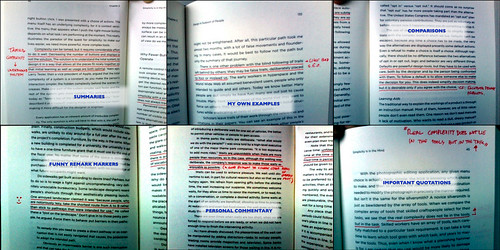
showing some notes I made while reading the book Living With Complexity, by Donald Norman. These notes fell into six cases:
Summaries of the arguments in text. Often texts will take long circuitous routes to get to the point. (Norman's book is not one of these.) I tend to write quick summaries, usually in implication form like the one above, that cut down the entropy.
My examples to complement the text. Sometimes I happen to know better examples, or examples that I prefer, than those in the book; in that case I tend to note them in the book so that the example is always connected to the context in which I thought of it. This is particularly useful in work books (and papers, of course) when I turn them into teaching or executive education materials.
Comparisons with external materials. In this case I make a note to compare Norman's point about default choices with the problems Facebook faced in similar matters regarding its privacy.
Notable passages. Marking funny passages with smiley faces and surprising passages with an exclamation point helps find these when browsing the book quickly. Occasionally I also mark passages for style or felicitous turn of phrase, typically with "nice!" on the margin.
Personal commentary. Sometimes the text provokes some reaction that I think is work recording in the book. I don't write review-like commentary in books as a general rule, but I might note something about missing or hidden assumptions, innumeracy, biases, statistical issues; I might also comment positively on an idea, for example, that I had never thought of except for the text.
Quotable passages. These are self-explanatory and particularly easy to make on eBooks. Here's one from George Orwell's Homage To Catalonia:
The constant come-and-go of troops had reduced the village to a state of unspeakable filth. It did not possess and never had possessed such a thing as a lavatory or a drain of any kind, and there was not a square yard anywhere where you could tread without watching your step. (Chapter 2.)
A few other types of marginalia that I have used in other books:
Proofs and analysis to complement what's in the text. As an example, in a PNAS paper on predictions based on search, the authors call $\log(y) = \beta_0 + \beta_1 \log(x)$ a linear model, with the logarithms used to account for the skewness of the variables. I inserted a note that this is clearly a power law relationship, not a linear relationship, with the two steps of algebra that show $y = e^{\beta_0} \times x^{\beta_1}$, in case I happen to be distracted when I reread this paper and can't think through the baby math.
Adding missing references or checking the references (which sometime are incorrect, in which case I correct them). Yep, I'm an academic nerd at heart; but these are important, like a chain of custody for evidence or the provenance records for a work of art.
Diagrams clarifying complicated points. I do this in part because I like visual thinking and in part because if I ever need to present the material to an audience I'll have a starting point for visual support design.
Data that complements the text. Sometimes the text is dequantized and refers to a story for which data is available. I find that adding the data to the story helps me get a better perspective and also if I ever want to use the story I'll have the data there to make a better case.
Counter-arguments. Sometimes I disagree with the text, or at least with the lack of feasible counter-arguments (even when I agree with a position I don't like that the author presents the opposing points of view only in strawman form), so I write the counter-arguments in order to remind me that they exist and the presentation in the text doesn't do them justice.
Markers for things that I want to get. For example, while reading Ted Gioia's The History of Jazz, I marked several recordings that he mentions for acquisition; when reading technical papers I tend to mark the references I want to check; when reading reviews I tend to add things to wishlists (though I also prune these wishlists often).
HOW to make notes
A few practical points for writing marginalia:
Highlighters are not good for long-term notes. They either darken significantly, making it hard to read the highlighted text, or they fade, losing the highlight. I prefer underlining with a high contrast color for short sentences or segments or marking beginning and end of passages on the margin.
Margins are not the only place. I add free-standing inserts, usually in the form of large Post-Its or pieces of paper. Important management tip: write the page number the note refers to on the note.
Transcribing important notes to a searchable format (a text file on my laptop) makes it easy to find stuff later. This is one of the advantages of eBooks of the various types (Kindle, iBook, O'Reilly PDFs), making it easy to search notes and highlights.
Keeping a commonplace book of felicitous turns of phrase (the ones in the books and the ones I come up with) either in a file or on an old-style paper journal helps me become a better writer.
-- -- -- --
Note: This blog may become a little more varied in topics as I decided to write posts more often to practice writing for a general audience. After all, the best way to become a better writer is to write and let others see it. (No comments on the blog, but plenty of ones by email from people I know.)
Monday, December 12, 2011
How many possible topologies can a N-node network have?
Short answer, for an undirected network: $2^{N(N-1)/2}$.
Essentially the number of edges is $N(N-1)/2$ so the number of possible topologies is two raised to the number of edges, capturing every possible case where an edge can either be present or absent. For a directed network the number of edges is twice that of those in an undirected network so the number of possible topologies is the square (or just remove the $/2$ part from the formula above).
To show how quickly things get out of control, here are some numbers:
$N=1 \Rightarrow 1$ topology
$N=2 \Rightarrow 2$ topologies
$N=3 \Rightarrow 8$ topologies
$N=4 \Rightarrow 64$ topologies
$N=5 \Rightarrow 1024$ topologies
$N=6 \Rightarrow 32,768$ topologies
$N=7 \Rightarrow 2,097,152$ topologies
$N=8 \Rightarrow 268,435,456$ topologies
$N=9 \Rightarrow 68,719,476,736$ topologies
$N=10 \Rightarrow 35,184,372,088,832$ topologies
$N=20 \Rightarrow 1.5693 \times 10^{57}$ topologies
$N=30 \Rightarrow 8.8725 \times 10^{130}$ topologies
$N=40 \Rightarrow 6.3591 \times 10^{234}$ topologies
$N=50 \Rightarrow 5.7776 \times 10^{368}$ topologies
This is the reason why any serious analysis of a network requires the use of mathematical modeling and computer processing: our human brains are not equipped to deal with this kind of exploding complexity.
And for the visual learners, here's a graph denoting the pointlessness of trying to grasp network topologies "by hand" (note logarithmic vertical scale):
Essentially the number of edges is $N(N-1)/2$ so the number of possible topologies is two raised to the number of edges, capturing every possible case where an edge can either be present or absent. For a directed network the number of edges is twice that of those in an undirected network so the number of possible topologies is the square (or just remove the $/2$ part from the formula above).
To show how quickly things get out of control, here are some numbers:
$N=1 \Rightarrow 1$ topology
$N=2 \Rightarrow 2$ topologies
$N=3 \Rightarrow 8$ topologies
$N=4 \Rightarrow 64$ topologies
$N=5 \Rightarrow 1024$ topologies
$N=6 \Rightarrow 32,768$ topologies
$N=7 \Rightarrow 2,097,152$ topologies
$N=8 \Rightarrow 268,435,456$ topologies
$N=9 \Rightarrow 68,719,476,736$ topologies
$N=10 \Rightarrow 35,184,372,088,832$ topologies
$N=20 \Rightarrow 1.5693 \times 10^{57}$ topologies
$N=30 \Rightarrow 8.8725 \times 10^{130}$ topologies
$N=40 \Rightarrow 6.3591 \times 10^{234}$ topologies
$N=50 \Rightarrow 5.7776 \times 10^{368}$ topologies
This is the reason why any serious analysis of a network requires the use of mathematical modeling and computer processing: our human brains are not equipped to deal with this kind of exploding complexity.
And for the visual learners, here's a graph denoting the pointlessness of trying to grasp network topologies "by hand" (note logarithmic vertical scale):
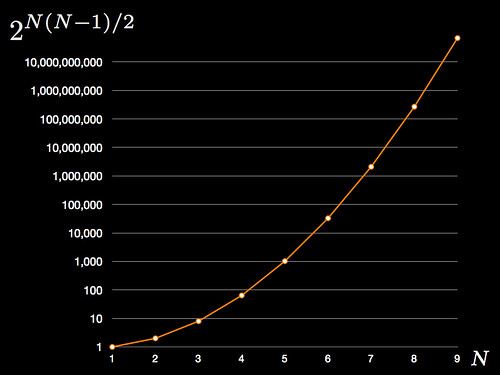
Saturday, December 3, 2011
Why I'm not a fan of "presentation training"
Because there are too many different types of presentation for any sort of abstract training to be effective. So "presentation training" ends up – at best – being "presentation software training."
Learning about information design, writing and general verbal communication, stage management and stage presence, and operation of software and tools used in presentations may help one become a better presenter. But, like in so many technical fields, all of these need some study of the foundations followed by a lot of field- and person-specific practice.
I recommend Edward Tufte's books (and seminar) for information design; Strunk and White's The Elements of Style, James Humes's Speak like Churchill, Stand like Lincoln, and William Zinsser's On Writing Well for verbal communication; and a quick read of the manual followed by exploration of the presentation software one uses. I have no recommendations regarding stage management and stage presence short of joining a theatre group, which is perhaps too much of a commitment for most presenters.
I have already written pretty much all I think about presentation preparation; the present post is about my dislike of "presentation training." To be clear, this is not about preparation for teaching or training to be an instructor. These, being specialized skills – and typically field-specific skills – are a different case.
Problem 1: Generic presentation training is unlikely to help any but the most incompetent of presenters
Since an effective presentation is one designed for its objective, within the norms of its field, targeted to its specific audience, and using the technical knowledge of its field, what use is it to learn generic rules, beyond the minimum of information design, clarity in verbal expression, and stage presence?
(My understanding from people who have attended presentation training is that there was little about information design, nothing about verbal expression, and just platitudes about stage presence.)
For someone who knows nothing about presentations and learns the basics of operating the software, presentation training may be of some use. I think Tufte made this argument: the great presenters won't be goaded into becoming "death by powerpoint" presenters just because they use the software; the terrible presenters will be forced to come up with some talking points, which may help their presentations be less disastrous. But the rest will become worse presenters by focussing on the software and some hackneyed rules – instead of the content of and the audience for the presentation.
Problem 2: Presentation trainers tend to be clueless about the needs of technical presentations
Or, the Norman Critique of the Tufte Table Argument, writ large.
The argument (which I wrote as point 1 in this post) is essentially that looking at a table, a formula, or a diagram as a presentation object – understanding its aesthetics, its information design, its use of color and type – is very different from looking at a table to make sense of the numbers therein, understand the implications of a formula to a mathematical or chemical model, and interpret the implications of the diagram for its field.
Tufte, in his attack on Powerpoint, talks about a table but focusses on its design, not how the numbers would be used, which is what prompted Donald Norman to write his critique; but, of all the people who could be said to be involved in presentation training, Tufte is actually the strongest advocate for content.
The fact remains that there's a very big difference between technical material which is used as a prop to illustrate some presentation device or technique to an audience which is mostly outside the technical field of the material and the same material being used to make a technical point to an audience of the appropriate technical field.
Presentation training, being generic, cannot give specific rules for a given field; but those rules are actually useful to anyone in the field who has questions about how to present something.
Problem 3: Presentation training actions are typically presentations (lectures), which is not an effective way to teach technical material
The best way to teach technical material is to have the students prepare by reading the foundations (or watching video on their own, allowing them to pace the delivery by their own learning speed) and preparing for a discussion or exercise applying what they learned.
This is called participant-centered learning; it's the way people learn technical material. Even in lecture courses the actual learning only happens when the students practice the material.
Almost all presentation training is done in lecture form, delivered as a presentation from the instructor with question-and-answer periods for the audience. But since the audience doesn't actually practice the material in the lecture, they may have only questions of clarification. The real questions that appear during actual practice don't come up during a lecture, and those are the questions that really need an answer.
Problem 4: Most presentation training is too narrowly bracketed
Because it's generic, presentation training misses the point of making a presentation to begin with.
After all, presentations aren't made in a vacuum: there's a purpose to the presentation (say, report market research to decision-makers), an audience with specific needs (product designers who need to understand the parameters of the consumer choice so they can tweak the product line), supporting material that may be used for further reference (a written report with the details of the research), action items and metrics for those items (follow-up research and a schedule of deliverables and budget), and other elements that depend on the presentation.
There's also the culture of the organization which hosts the presentation, disclosure and privacy issues, reliability of sources, and a host of matters apparently unrelated to a presentation that determine its success a lot more than the design of the slides.
In fact, the use of slides, or the idea of a speaker talking to an audience, is itself a constraint on the type of presentations the training is focussed on. And that trains people to think of a presentation as a lecture-style presentation. Many presentations are interactive, perhaps with the "presenter" taking the position of moderator or arbitrator; some presentations are made in roundtable fashion, as a discussion where the main presenter is one of many voices.
Some time ago, I summarized a broader view of a specific type of presentation event (data scientists presenting results to managers) in this diagram, illustrating why and how I thought data scientists should take more care with presentation design (click for larger):
(Note that this is specific advice for people making presentations based on data analysis to managers or decision-makers that rely on the data analysis for action, but cannot do the analysis themselves. Hence the blue rules on the right to minimize the miscommunication between the people from two different fields. This is what I mean by field-specific presentation training.)
These are four reasons why I don't like generic presentation training. Really it's just one: generic presentation training assumes that content is something secondary, and that assumption is the reason why we see so many bad presentations to begin with.
NOTE: Participant-centered learning is a general term for using the class time for discussion and exercises, not necessarily for the Harvard Case Method, which is one form of participant-centered learning.
Related posts:
Posts on presentations in my personal blog.
Posts on teaching in my personal blog.
Posts on presentations in this blog.
My 3500-word post on preparing presentations.
Learning about information design, writing and general verbal communication, stage management and stage presence, and operation of software and tools used in presentations may help one become a better presenter. But, like in so many technical fields, all of these need some study of the foundations followed by a lot of field- and person-specific practice.
I recommend Edward Tufte's books (and seminar) for information design; Strunk and White's The Elements of Style, James Humes's Speak like Churchill, Stand like Lincoln, and William Zinsser's On Writing Well for verbal communication; and a quick read of the manual followed by exploration of the presentation software one uses. I have no recommendations regarding stage management and stage presence short of joining a theatre group, which is perhaps too much of a commitment for most presenters.
I have already written pretty much all I think about presentation preparation; the present post is about my dislike of "presentation training." To be clear, this is not about preparation for teaching or training to be an instructor. These, being specialized skills – and typically field-specific skills – are a different case.
Problem 1: Generic presentation training is unlikely to help any but the most incompetent of presenters
Since an effective presentation is one designed for its objective, within the norms of its field, targeted to its specific audience, and using the technical knowledge of its field, what use is it to learn generic rules, beyond the minimum of information design, clarity in verbal expression, and stage presence?
(My understanding from people who have attended presentation training is that there was little about information design, nothing about verbal expression, and just platitudes about stage presence.)
For someone who knows nothing about presentations and learns the basics of operating the software, presentation training may be of some use. I think Tufte made this argument: the great presenters won't be goaded into becoming "death by powerpoint" presenters just because they use the software; the terrible presenters will be forced to come up with some talking points, which may help their presentations be less disastrous. But the rest will become worse presenters by focussing on the software and some hackneyed rules – instead of the content of and the audience for the presentation.
Problem 2: Presentation trainers tend to be clueless about the needs of technical presentations
Or, the Norman Critique of the Tufte Table Argument, writ large.
The argument (which I wrote as point 1 in this post) is essentially that looking at a table, a formula, or a diagram as a presentation object – understanding its aesthetics, its information design, its use of color and type – is very different from looking at a table to make sense of the numbers therein, understand the implications of a formula to a mathematical or chemical model, and interpret the implications of the diagram for its field.
Tufte, in his attack on Powerpoint, talks about a table but focusses on its design, not how the numbers would be used, which is what prompted Donald Norman to write his critique; but, of all the people who could be said to be involved in presentation training, Tufte is actually the strongest advocate for content.
The fact remains that there's a very big difference between technical material which is used as a prop to illustrate some presentation device or technique to an audience which is mostly outside the technical field of the material and the same material being used to make a technical point to an audience of the appropriate technical field.
Presentation training, being generic, cannot give specific rules for a given field; but those rules are actually useful to anyone in the field who has questions about how to present something.
Problem 3: Presentation training actions are typically presentations (lectures), which is not an effective way to teach technical material
The best way to teach technical material is to have the students prepare by reading the foundations (or watching video on their own, allowing them to pace the delivery by their own learning speed) and preparing for a discussion or exercise applying what they learned.
This is called participant-centered learning; it's the way people learn technical material. Even in lecture courses the actual learning only happens when the students practice the material.
Almost all presentation training is done in lecture form, delivered as a presentation from the instructor with question-and-answer periods for the audience. But since the audience doesn't actually practice the material in the lecture, they may have only questions of clarification. The real questions that appear during actual practice don't come up during a lecture, and those are the questions that really need an answer.
Problem 4: Most presentation training is too narrowly bracketed
Because it's generic, presentation training misses the point of making a presentation to begin with.
After all, presentations aren't made in a vacuum: there's a purpose to the presentation (say, report market research to decision-makers), an audience with specific needs (product designers who need to understand the parameters of the consumer choice so they can tweak the product line), supporting material that may be used for further reference (a written report with the details of the research), action items and metrics for those items (follow-up research and a schedule of deliverables and budget), and other elements that depend on the presentation.
There's also the culture of the organization which hosts the presentation, disclosure and privacy issues, reliability of sources, and a host of matters apparently unrelated to a presentation that determine its success a lot more than the design of the slides.
In fact, the use of slides, or the idea of a speaker talking to an audience, is itself a constraint on the type of presentations the training is focussed on. And that trains people to think of a presentation as a lecture-style presentation. Many presentations are interactive, perhaps with the "presenter" taking the position of moderator or arbitrator; some presentations are made in roundtable fashion, as a discussion where the main presenter is one of many voices.
Some time ago, I summarized a broader view of a specific type of presentation event (data scientists presenting results to managers) in this diagram, illustrating why and how I thought data scientists should take more care with presentation design (click for larger):
(Note that this is specific advice for people making presentations based on data analysis to managers or decision-makers that rely on the data analysis for action, but cannot do the analysis themselves. Hence the blue rules on the right to minimize the miscommunication between the people from two different fields. This is what I mean by field-specific presentation training.)
These are four reasons why I don't like generic presentation training. Really it's just one: generic presentation training assumes that content is something secondary, and that assumption is the reason why we see so many bad presentations to begin with.
NOTE: Participant-centered learning is a general term for using the class time for discussion and exercises, not necessarily for the Harvard Case Method, which is one form of participant-centered learning.
Related posts:
Posts on presentations in my personal blog.
Posts on teaching in my personal blog.
Posts on presentations in this blog.
My 3500-word post on preparing presentations.
Labels:
presentations,
teaching
Friday, December 2, 2011
Dilbert gets the Correlation-Causation difference wrong
This was the Dilbert comic strip for Nov. 28, 2011:
It seems to imply that even though there's a correlation between the pointy-haired boss leaving Dilbert's cubicle and receiving an anonymous email about the worst boss in the world, there's no causation.
THAT IS WRONG!
Clearly there's causation: PHB leaves Dilbert's cubicle, which causes Wally to send the anonymous email. PHB's implication that he thinks Dilbert sends the email is wrong, but that doesn't mean that the correlation he noticed isn't in this case created by a causal link between leaving Dilbert's cubicle and getting the email.
I think Edward Tufte once said that the statement "correlation is not causation" was incomplete; at least it should read "correlation is not causation, but it sure hints at some relationship that must be investigated further." Or words to that effect.
It seems to imply that even though there's a correlation between the pointy-haired boss leaving Dilbert's cubicle and receiving an anonymous email about the worst boss in the world, there's no causation.
THAT IS WRONG!
Clearly there's causation: PHB leaves Dilbert's cubicle, which causes Wally to send the anonymous email. PHB's implication that he thinks Dilbert sends the email is wrong, but that doesn't mean that the correlation he noticed isn't in this case created by a causal link between leaving Dilbert's cubicle and getting the email.
I think Edward Tufte once said that the statement "correlation is not causation" was incomplete; at least it should read "correlation is not causation, but it sure hints at some relationship that must be investigated further." Or words to that effect.
Labels:
causality,
Dilbert,
Probability,
statistics
Subscribe to:
Posts (Atom)